Season 3: AI for Good Medicine
How do we envision artificial intelligence (AI), machine learning (ML), or any other deep learning technology delivering good medicine for all? The healthcare industry cannot embrace the next frontier of medicine if it is not pragmatic, responsible, and equitably valuable. Can these deep learning technologies make a real and trusted impact on improving outcomes for patients anywhere from drug development to healthcare delivery?
Episode 1
The Balance: AI's Healthcare Goodness for Marginalized Patients
Can Artificial Intelligence (AI) and Machine Learning (ML) support fairness, personalization, and inclusiveness to chip away at the epidemic of healthcare inequity, or will it further create more inequity in the healthcare system?
Speaker
Sampath Veeraraghavan
Sampath Veeraraghavan is a globally renowned technologist best known for his technological innovations in addressing global humanitarian and sustainable development challenges. He is a seasoned technology and business leader with over 17 years of experience in the Top 500 Fortune companies. Throughout his career, he has led business-critical strategic R & D programs and successfully delivered cutting-edge technologies in areas of Conversational Artificial Intelligence (AI), Natural Language Understanding, Cloud computing, Data privacy, Enterprise systems, Infrastructure technologies, Assistive and Sustainable technologies. Sampath served as an expert in the 2020 Broadband Commission working group on school connectivity co-chaired by UNESCO, UNICEF, and ITU to drive “GIGA,” a Global School Connectivity Initiative. He is the founder and president of “The Brahmam,” a humanitarian program delivering next-generation social innovations to achieve sustainable development goals and benefit marginalized communities globally. Over a decade, he has launched large-scale transformational global initiatives that brought together academic institutions, industry leaders, and Government agencies to address pressing global challenges faced by children with disabilities, impoverished women, and students from marginalized communities in developing nations.
Sampath serves as the Global Chair of the 2021 -2022 IEEE Humanitarian Activities Committee (IEEE HAC) of the world’s largest technical professional organization “The Institute of Electrical and Electronics Engineers (IEEE), USA. In this role, he spearheads the global strategy and portfolio of sustainable development and humanitarian engineering programs to deliver impactful programs to engage and benefit 400K+ IEEE members at the grassroots in 160 countries. He is credited with launching several novel global programs in humanitarian engineering which successfully inspired and engaged students and young professionals in sustainable development activities globally. Sampath was the Global Chair (2019-2020) of IEEE Special Interest Group on Humanitarian Technologies (SIGHT), leading the program to record-breaking growth through high-impact, technology-driven sustainable programs benefiting members in 125+ countries. He is the founding chair for the IEEE SIGHT day (2020), SIGHT week (2019), and the inaugural IEEE Global HAC summit (2021), a portfolio of global programs that showcases the impactful IEEE technology-based humanitarian programs. He currently chairs the IEEE Standard’s 2021-2022 corporate sustainability global efforts. As an active IEEE and IEEE-HKN member, Sampath has spearheaded more than 20+ global committees and has made significant contributions in advancing technology for the benefit of humanity. Sampath is the 2022 president-elect of the IEEE Eta Kappa Nu (IEEE-HKN), one of the world’s topmost honor societies in science and technology established in 1904 with over 200,000 life members and 260 global chapters. This makes him one of the youngest presidents in the history of IEEE-HKN.
Sampath is honored with numerous (15+) global awards for his leadership and technical excellence in delivering innovative technologies and global programs to address the humanitarian and sustainable development challenges. He was recently honored with one of the top global awards “the 2020 IEEE Theodore W. Hissey Professional Award”. He has delivered 300+ invited talks in International forums, premier technology conferences, and industry panels organized by UN, IEEE, ITU, World IoT forum, and Top universities around the globe. He has authored and published 30+ research publications and thought leadership articles in leading global conferences, journals, and magazines.
His technological innovations and leadership excellence were featured in cover stories of global media such as IEEE TV, IEEE spectrum, USA today, E-week, AI-news, IEEE Institute, and IEEE transmitter, The Bridge, and ACM-News. He received an M.S. degree in Electrical Engineering from Tufts University, Massachusetts, USA (2010) and a B.E. degree in Computer Science and Engineering from Anna University, India (2005). He is accredited with leading and delivering some of the industry-first programs in Artificial intelligence and computing technologies across multi-disciplinary domains. He currently works as a senior technology and program management leader in the conversational Artificial Intelligence industry where he spearheads a portfolio of science and engineering programs to advance spoken language innovations.
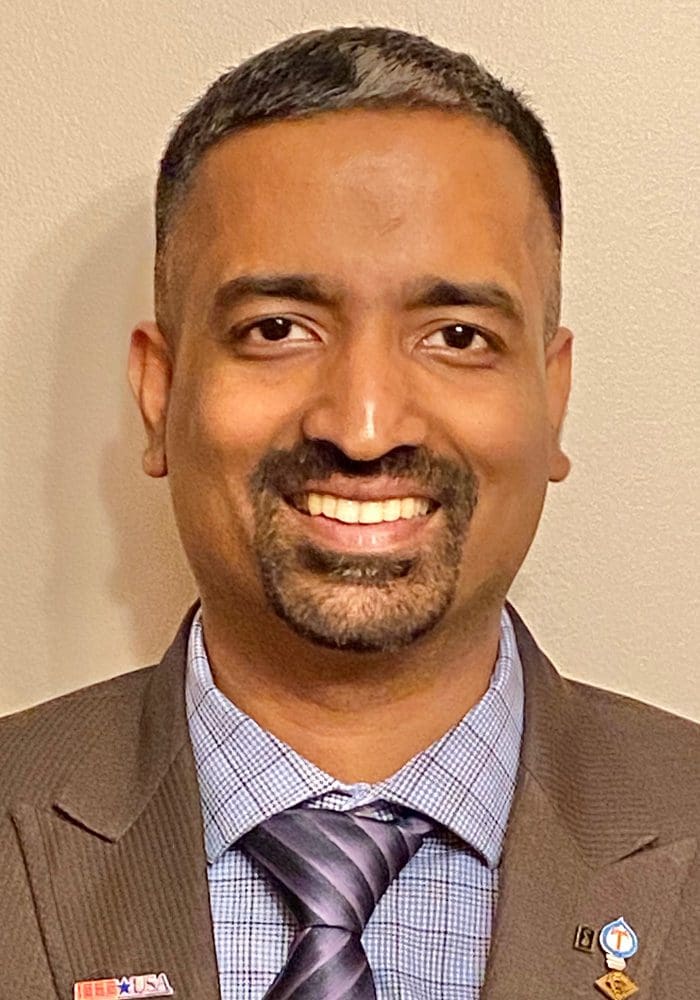
Full Transcript
Maria Palombini
Hello everyone! I am Maria Palombini, and I’m with the IEEE Healthcare & Life Sciences Practice. Today, we’re kicking off Season 3 of the Re-Think Health Podcast Series. Season 3, will focus on AI for Good Medicine. So a little bit about the Re-Think Health Podcast Series. We talk to multidisciplinary experts around the world, focused on various themes and topics, and we want to bring awareness and balance understanding in all these new technologies, tools, and applications, where we may need some policies or standards, all in the name of driving responsible, trusted adoption to give better health for all. We have previous seasons on Podbean, iTunes, and you can learn more.
So AI for Good Medicine, which is the focus of our theme on this Season 3, will bring again more multidisciplinary experts from around the globe to really answer or provide insight into questions is how do we invision Artificial Intelligence, Machine Learning or other deep learning technologies to deliver good medicine for all, right?
We all want good medicine, but at what price? Price, meaning in terms of trust and validation in its use. We are not looking for the next frontier of medicine if it’s not pragmatic, if it’s not responsible and can be equitably valuable to all. So in this season, we go directly to the technologists, clinicians, researchers, ethicists, regulators, and others, and talk about how these deep learning technologies can make real and trusted impact on improving outcomes for patients anywhere from drug development to healthcare delivery.
Here’s the question. Will the AI, the Machine Learning, the deep learning cut through the health data swamp for better health outcomes? So with that, I would like to welcome Sampath Veeraraghavan to our discussion on the true potential of AI in healthcare and helping marginalized populations. This has become a critical topic for debate, and he’s going to be helping this with us.
Welcome Sampath.
Sampath Veeraraghavan
Hey Maria, wonderful to join you all here on this podcast.
Maria Palombini
Thank you so much. So before we get to the details of the technology, the applications, the debate at hand, I like to humanize the experience. So a little bit about Sampath. He’s the Global Chair at the IEEE Humanitarian Activities Committee, or sometimes here we call it the HAC. He’s President Elect for IEEE Eta Kappa Nu and the 2020 recipient of the IEEE Ted Hissey Outstanding Young Professionals Award. He has more than 17 years of research and industrial experience in spearheading business critical strategic R&D programs and has successfully delivered cutting-edge technologies in areas of conversational Artificial Intelligence, natural language understanding, cloud computing, assistive and sustainable technologies. He’s globally best known for his technological innovations in addressing global humanitarian and sustainable development challenges. And this is why he is the first person and the most important person to talk to about this critical debate. First of all, Sampath, tell us about your work and objectives at the IEEE HAC and now with this new thing coming up with Eta Kappa Nu.
Sampath Veeraraghavan
Thank you Maria. First, as the Global Chair of IEEE Humanitarian Activities Committee, also called as, HAC, I lead the overall strategy and the portfolio of global programs, primarily focused on inspiring, connecting, and engaging close to half a million IEEE members. So they can take and apply the technical skills for social good.
We primarily focus on four major strategic areas as part of our work. The first one is raising awareness for technologists on how you can put the technical skills for addressing the grand challenges in the sustainable development space. Second, we provide the educational materials and training so that IEEE members could advance their skills for social good engagement. Third, we also provide project funding program so that we could actually empower our members transform their ideas into actionable projects, which could address the local challenges.
And lastly, we want to foster a global ecosystem to bring together technologists, local community, and partners so that we could address pressing global challenges. And our efforts strongly aligns with IEEE’s core mission and are critical to achieving IEEE’s strategic goals. And in fact, in 2021, I know I was very delighted to spearhead the HAC to a record breaking group.
Since its inception where we achieved a record of more than 25,000 site members and we received close to 385 projects globally and supported close to a hundred projects and we established 30 plus global partnerships and launched key programs- IEEE HAC Global Summit, positioning IEEE as leader in the sustainable development space.
Speaking about IEEE Eta Kappa Nu, it’s one of the world’s oldest, top-most honors society in science and engineering. And about 10 years back, it was merged with IEEE and it has about close to 200,000 life members throughout the world. And it has more than 200 chapters around the world. Many pioneers in the technology industry are part of Eta Kappa Nu.
So really excited to lead this true, true global impact program that has a true impact at our members at grassroots.
Maria Palombini
Absolutely. I know you’ve been doing great work at the HAC and, I’ve talked to other volunteers who work with you. They’re super excited and obviously great overall for our organization globally.
You know, Sampath, you have such a diverse background, so much research technology. What inspired you? What ignited your passion to really look into where technology can be used for good?
Sampath Veeraraghavan
Thank you, Maria. That’s a very important question. In fact, to answer this question, I have to do a time travel. I have to go back almost 18 years, back in my journey/career. So my journey actually started as a student in India, where I leveraged computing technologies to design an automated screening system to detect development delays in children. Basically this effort, that opened up critical opportunities to initiate early intervention programs, to treat children with special needs like autism, benefiting families below the poverty line in Southern India.
This, I think, was a very major stepping stone in terms of my inspiration because this program helped me to directly connect with the marginalized population, see how I could, as a technologist, come back with an appropriate, relevant and low-cost solution to address their needs. So the success of this effort made me realize how it is very important for technologists, especially like engineers and IEEE members, to apply your technical skills and leadership skills for social good.
This further ignited my passion and inspired me to start the humanitarian engineering program called as Brahmam, meaning knowledge. Which aims to deliver next generation social innovations to serve the needs of marginalized communities. This has been a very amazing journey in the last 17 to 18 years, where I have launched several global initiatives that are brought to the academic institution, industry leaders, our professional organizations, and governmental agencies to address pressing global challenges faced by children with disabilities, impoverished women, and students from marginalized communities in developing nations.
Maria Palombini
Wow. That’s such great work and such important work. And I could see how it’s carried you all the way to what you’re doing now. Now we’re going to get a little more deeper into the technology and to the discussion around this concept of AI for Good Medicine and for the audience out there. When I say AI, I’m not exclusively talking about artificial intelligence, but the whole realm of machine learning, deep learning technologies in AI.
So, Sampath, maybe you can just explain a little bit to our audience, the kind of research exposure or hands-on tech development that you’ve had around these types of technologies in health applications that you may have experienced firsthand.
Sampath Veeraraghavan
Thank you, Maria. That’s a great question. So throughout my career, I have spearheaded large-scale transformational AI programs in healthcare. Before I touch upon some examples, it’s important to understand some of the AI core capabilities like machine learning, computer vision, natural language understanding, and speech recognition. All of these capabilities offers new approaches to solve the toughest challenges in healthcare. For instance, machine learning techniques like deep learning, it offers the powerful capability to create sophisticated models that can be leveraged for a wide variety of healthcare use cases, prediction, forecasting, classification, so forth. Similarly, computer vision techniques, it processes the visual information in detail, in images and videos to generate valuable influences.
If you leverage the AI model along with these advanced techniques, it helps us to create solutions and tools that can assist medical practitioners to examine clinical images, identify hidden patterns of tumors and which in turn supports the expidited decision-making and delivering an effective plan for patients. Specifically as part of my career journey, there are two major programs I would like to call out.
I lead a major initiative called as Information Systems on Human and Health Services. Essentially, this is a first of its kind system, which was focused on tracking the statewide visibility in Southern India.
What we did was we collected all the statewide permissions. We leveraged machine learning techniques to process the massive amount of data and interconnected the underlying patterns, and very meaningful insights to support decision-making. And this in turn empowered the healthcare providers, policymakers, governmental agencies, and disabled individuals to understand the disability prevalence pattern and initiate prevention measures. And also it helps them to better understand the needs of disabled citizens and facilitated the creation of equal opportunities for them, both in terms of education and employment.
The second major project I would like to call out here is on the Conversational AI Spectrum. Recently I was involved in delivering one of the industry’s first programs in conversational AI technologies to advance voice innovations for the healthcare industry. So the idea here is we wanted to expand the conversational AI capabilities so that we could support healthcare use cases like prescription ordering and urgent care appointments. Again, all this AI core capability, what we touched upon, it all offers a very unique and universal approach in opening up critical opportunities to further drive AI innovation in the enterprise healthcare segment, actually.
Maria Palombini
That’s fascinating, Sampath. I think that’s so much great work you continue to demonstrate. It’s unbelievable and great for all of humanity. I like to do this with my interviewees. I say to them think quick. So when I say to you, or you hear the term “AI for Good Medicine,” what comes to mind and why?
Sampath Veeraraghavan
Thank you, Maria. AI can be used to handle some of the greatest challenges in the healthcare segment. When we talk about AI for Good Medicine, few things come to my mind. One is how do you creatively use machine learning models to leverage and handle voluminous amount of medical data and uncover insights to help improve health outcomes and patient experiences. Specifically, there are a couple of areas that come to my mind where I feel creatively, we could play an important role to solve some key challenges.
First, how do we accelerate medical researchers to advance the prevention, diagnosis, and treatment of diseases, right? And increasingly AI models becomes important in this segment where we are empowering healthcare providers with right tools. Today, the doctors don’t have to scan through thousands of CT majors. They rather could use an automated AI system to help them to identify the most important ones so that they could expedite their decision-making. Similarly, the second important area that comes to my mind is reducing the health inequity and improving access to care for underserved population. This is important because, as you see, 50% are not connected to the internet. So the goal is how do we devise solutions, which can work both real time and offline? At the same time, provides a robust approach to simplify the access to health care and also to provide a critical opportunity to bring these people to the mainstream healthcare services.
Thirdly, AI today is vital. You rightly said we need to think about innovations in patient-centered innovations. We are no longer in the age of mass production. We are living in the age of mass personalization. So I think when we talk about AI for Good Medicine, this is also key because today the AI is empowering the healthcare providers to see if there’s a rare condition. It helps them to closely understand what are the other similar patterns they have seen in other patients and helps them to customize based on the genetic condition how they could help the current patient.
And lastly, it’s also important that we have ethical and responsible use of AI to save all patient’s privacy. So these are some broader, a couple of things that comes to my mind, but I think the most important thing is, as I said, the ability to handle voluminous amount of data that transforms the opportunity to provide low-cost solutions. I think that’s going to be key in terms of achieving and supporting the overall good, wellbeing of the larger community.
Maria Palombini
Absolutely. I think that AI has a lot of promising opportunities, but we’re going to get a little bit into maybe its challenges in just a little bit.
This is the core of our discussion with them about technology for good and equitable access. So, I’ve heard the argument that perhaps AI machine learning can support fairness, personalization, and inclusiveness in healthcare helping to address the healthcare inequity question. In your opinion, in all the work you’re doing, do you find that AI can actually help address the racial, gender, and socioeconomic disparities in healthcare systems? Or, like some have argued, that it further creates more inequity in the healthcare system.
Sampath Veeraraghavan
Thank you, Maria. It’s a challenging question. I think the potential of AI and the challenges of AI are equally big in my view. So the answer is yes/yes to both, actually. Let’s first look at some of the potential. What are the possibilities, right?
Now, if you look at the super power of these AI systems, they can look through large amounts of data and it can help us to surface the right information or the right prediction at the right level to the right stakeholder. This is going to be very important because now with the advancements in cloud computing, and capabilities like a deep learning models, it’s going to help us to drive the next frontiers of innovation that will empower healthcare stakeholders with tools to compare patient cases to every other patient who ever had the same kind of disease or pattern. It results in a data-driven approach to identify the most effective treatment. And that’s one best-suited for the specific genetic subtype of the disease in someone with a certain genetic background.
That’s truly personalized medicine. That’s truly a personalized approach in digital healthcare and the prognosis should also be good, actually. And now, if you think from the same angle, this can also empower people living in poor settings. We can leverage automated chatbots that potentially could help us to screen for some symptoms, so that it can reduce the burden of the medical teams in those setups, actually. So there’s a ton of super power and possibilities here, how AI could actually help to address the racial, gender, and socioeconomic disparity in healthcare systems.
With that said it also comes with its own potential risk. For instance, a poorly designed a system can be misdiagnosed, right? And that’s going to be a trust-breaker and the impact is going to be even more larger. And similarly, remember these systems are heavily dependent on data upon which they are trained on. So that means if there is a cultural bias within this dataset that, unfortunately, will be incorporated into the system and those blind spots will be integrated into the environment where they’re deployed. One of the challenges I also see here as certain problems, you also require high quality data where you could actually can get a very robust AI model. If that data, which you used is, again, biased or otherwise flawed, it’s going to be reflected in the performance of your system.
And lastly, we spoke only about the data, but also remember the AI system also has something called as algorithms. So if the developer is unaware of the unintentional bias and introduces that bias in this programmatic logic, that’s also going to maximize discrimination.
So that’s why it’s important that technologists looking at developing AI-based solutions, you have to make sure that you proactively have measures in place where you have to maximize your impact and minimize the risk in terms of disparity creation. So I think the answer is, AI has tremendous potential, but also it comes with this risk. So it’s very important that we put controls in place to limit the negative impact of technology.
Maria Palombini
Absolutely. And you just basically answered by next question. You just jumped in front of it. I think that everything goes hand in hand. We can’t just think about benefits and opportunities of anything without thinking of the challenges that comes with it.
When you think of this whole thing, right? All these, this technology groundbreaking, that kind of thing- what’s the single most challenging aspect or gap? By gap, it could be security, lack of open data, lack of standards, not the right policy written, whatever that might be is currently not in AI applications or deep learning or machine learning, what have you, that continues to cause concern or uncertainty in credibility and trust of tools in this healthcare application? In your opinion, what is it? What could it be? Or I’m sure there’s more than one , maybe. And where do you think is the best path in resolving it?
Sampath Veeraraghavan
Thank you, Maria. It’s an important question, but again, there are several pieces that’s integrated here. You have rightly called out several dimensions here, right? Security, privacy, open data, lack of standards. So if you have to answer this question, I think I’m going to take one step back and give you a holistic dimension.
First of all, it’s very important to understand that AI is not a “one size fits all” approach. So I think it’s important for the global community to know that AI solutions are here to supplement. To reduce the pain point experienced with stakeholders in healthcare. It’s also critical to understand that these systems adapt over time because you are not deploying an algorithm in vacuum. You are actually deploying a technology, which is going to be part of an environment, but people will track with it and the system will adapt to it. For instance, if it designed some kind of scoring system today to rank a medical device or a solution it’s going to change, actually. So in my view, I think the key important challenge right now is we need to actually create a global ecosystem where we bring together policymakers, technologists, the local communities, and healthcare professionals, to holistically work together, to define standards along the lines of security, privacy, open data access, and so forth. We need to develop cost-effective AI models and products that can empower physicians and practices and hospitals to incorporate AI into daily clinical use. I think we should make sure there’s awareness and there’s also from technology standpoint, we are working from patient-centered innovations so that AI is seen as a complimentary technology to empower them rather than being seen as human machines.
There are also a few important things we need to do as part of this ecosystem. For example, the responsible use of AI. This can be achieved by enforcing standards and best practices to implement fairness, inclusiveness, security, and privacy controls. A few examples here is you could always check as a technologist, whether your models and datasets for bias and negative experiences. And there are several techniques in the industry like data visualization and clustering, which can evaluate the datasets distribution for fair representation of various stakeholders dimensions.
Secondly, you can also do routine updates to your training and testing datasets, which are essential to fairly account for diversity in users’ growing needs and usage pattern. And of course, they’ve got the sensitive user information for their patients which need privacy controls, like encrypting data at rest or in transmit.
So by doing all those things and also having a retention policy in place, you’re kind of making sure that you’re not only doing the right thing and you’re also working backwards, by focusing more on what the patients want and what the doctor needs and devise solutions, which are truly specific, relevant, actionable, and impactful.
So in a nutshell, we need a global ecosystem. At the same time, this ecosystem should provide standards and framework, which will enable us to develop universal solutions which can be easily developed and deployed whether it’s a developing nation or in an underdeveloped nation set up. I think we really need to have good quality data, high quality standards, and an interoperable framework where technologists can develop plug and play solutions, which can help us to support large scale and easily deployable solutions.
Maria Palombini
Wow. That’s very important. I think the idea of the plug and play and interoperability and all this- there’s so many questions and challenges around all these technologies. Something to have our audience take away with. You’ve already shared so much insight, so many great ideas and opportunities and things to think about.
Any final thoughts that you’d like to share with our audience- a call to action, something that they should look into, an opportunity that may be of interest to them of getting involved?
Sampath Veeraraghavan
I think the one important out here is first of all, huge kudos to IEEE Standards and to you for starting this podcast, because the timing of this podcast series and the thematic areas is very important given that we are still trying to come out of the pandemic situation.
Now, in terms of the final thought, I think AI tools has played a very tremendous role, especially in the pandemic situation, like COVID. Many places throughout the world help us to deliver solutions to track the pandemic, forecast the demand and supply, helping the local governmental agencies and healthcare.
There are two aspects to it, right? One, I think as a technologist, how you can advance these innovations in AI and second, most important thing is what does your local community need? I think it’s very important when the need and the technology meets that actually decides the bigger innovation.
And that’s where IEEE comes into play, they provide a tremendous amount of opportunity. For example, IEEE Humanitarian Activities Committee, as I told you earlier, we provide a portfolio of programs for you to participate, particularly with technical skills for social good. So definitely participate in these programs.
And again, IEEE Standards is an important program. Most recently we have launched Patient-centered Healthcare System Virtual Pitch Competition, which is also very important. You are able to mentor and guide these teams, I think that’s also a way for you to give back to the society.
I think I’m going to quote here, Leonardo da Vinci, the famous quote: “I have been impressed with the urgency of doing. Knowing is not enough; we must apply. Being willing is not enough; we must do.”
So I think we need to build inclusive and prosperous futures for everyone. I think it’s important, as a technologist, we all should look for an avenue to apply our technical and leadership skills for the larger good, so that we can collectively advance technology for the benefit of humanity.
Maria Palombini
If you want to learn more about the IEEE Humanitarian Activities Committee, you can visit hac.ieee.org. If you didn’t get that, you can visit the blog posts. We have links to activities Sampath is affiliated with plus other activities. In addition, just to let you all know that Sampath served as Advisory Committee Member and a Judge on the recent IEEE Re-think the Machine: Transforming RPM into a Patient-centered Healthcare System Virtual Pitch Competition, which aired on February 8th, 2022.
The HAC is actually going to mentor the first place winner student category for, hopefully, a potential pilot of their solution in the remote patient monitoring space. All of this information is available on the blog post affiliated with this podcast. So as you can see, a lot of the conversation we had today, the concepts that Sampath shared with us are addressed in various activities within our Healthcare and Life Science practice.
You know, the whole season is really going to get into these important themes- the benefits, the opportunities, but as well as the challenges that we can’t just neglect. And so when we come to these unsolved questions, this is what we bring a global community to collaborate, identify, explore, and build solutions in the form of tech and data standards, to really address some of these questions that are inhibiting the industry from moving forward and fully embracing the technologies.
So with that, I invite all of you if you’re interested to get involved in any of the work, as well as the HAC, or any of the work here at the Healthcare & Life Science practice, you can visit the practice website at ieeesa.io/hls. And hopefully, you can come along and join us for this great global experience.
I want to say a special thank you to Sampath for joining us today.
Sampath Veeraraghavan
Thank you, Maria.
Maria Palombini
And to our audience for tuning in. I wish all of you to continue to stay safe and well, and hopefully join us next time. Until then, take care.
Episode 2
Riding the Third Wave of AI for Precision Oncology
Hear about the recently released case study on the application of the “third wave of AI” that offers real-world data and practice on realizing the potential for precision oncology.
Speakers
Anthoula Lazaris
Scientific Director, McGill University Health Centre (MUHC) Research Institute
Anthoula Lazaris is a scientist with over 26 years combined experience in academia (McGill University) and biotechnology/industry, in management and senior-level positions with a demonstrated history of working in the hospital & healthcare industry. Lazaris possesses strong, technical skills in the areas of molecular biology, cell biology, genetically modified organisms (animals, mammalian cells, fungi, and bacteria), gene therapy, and recombinant protein production.
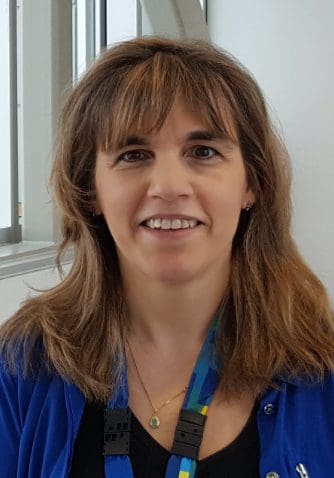
Nathan Hayes
CEO & Co-Founder, Modal Technology Corporation
Nathan Hayes is an entrepreneur, mathematician, and software architect with more than 20 years of experience working in these combined fields. Hayes specializes in the applied science of modal interval analysis to the fields of artificial intelligence, machine learning, and high-performance computing.
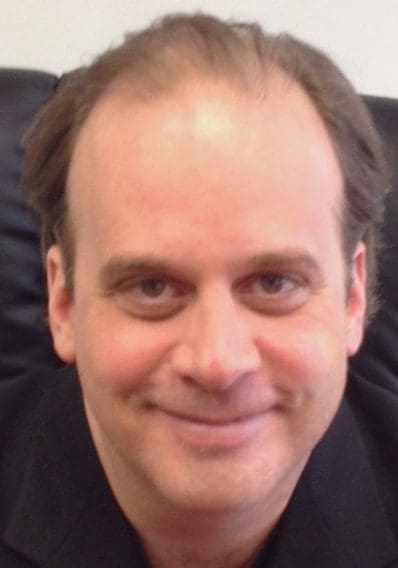
Full Transcript
Maria Palombini
Hello everyone! I am Maria Palombini, and I am Director and Lead of the Healthcare and Life Sciences practice here at the IEEE SA. And I’m also your host for the Re-think Health Podcast, Season 3: AI for Good Medicine.
The Healthcare Life Science practice is a platform for bringing multidisciplinary stakeholders from around the globe to collaborate, explore, and develop solutions that will drive responsible adoption of new technologies and applications leading to more security protection, and sustainable equitable access to quality of care for all individuals. Yes, this is an ambitious goal, but a much necessary one. The Re-think Health Podcast series seeks to bring awareness of these new technologies and applications with a balanced understanding in how to use them, how to be applied, and where potentially they may be need for policy standards or whatever it takes to drive more trusted and validated adoption to enable better health for all.
We have previous seasons available on Podbean, iTunes, or your favorite podcast provider. AI for Good Medicine Season 3 will bring a suite of multidisciplinary experts, technologists, clinicians, regulators, researchers, all with the goal to provide insights as how do we envision artificial intelligence or machine learning or any other deep learning technology, delivering good medicine for all? Naturally, we all want good medicine, but at what price? Especially, in terms of trust and validation and its use. So as healthcare industry stakeholders, we are not looking for the next frontier of medicine if it’s not pragmatic, responsible, and can be equitably valuable. So in this season, we go directly to all these experts and we try to get to the bottom of it and make real and trusted impact improving outcomes for patients anywhere from drug development to healthcare delivery.
So the question is: will AI, ML, deep learning cut-through the health data swamp for better health outcomes? With that, I would like to welcome Anthoula Lazaris, Scientist at the Research Institute of McGill University Health Center, and Nate Hayes, Founder and CEO of Modal Technology Corp. In this discussion, they’re going to talk to us about the third wave of AI for better patient outcomes and potentially realizing precision oncology. This is a fascinating case study. From the minute I heard about it, I was very excited about it, and I think it really shows how we can start to move the needle forward.
We are now on segment 1. We like to humanize the experience for our audience and we want to humanize the people behind the microphones. So a little bit about Anthoula. She has more than 26 years combined experience in academia, McGill University, biotechnology industry and management in senior-level positions with a demonstrated history of working in the hospital in the healthcare industry. She has been at the Research Institute of McGill University Health Center for a little over 11 years, focusing on bringing precision oncology to patients through clinical research projects.
Some of her career highlights include being part of the team making Nexia’s IPO, the largest public offering in life sciences in Canada, up to 2002. She was the first to demonstrate that a translation initiation factor can act as a proto-oncogene. The work was published in Nature.
And, Nate. He’s an entrepreneur, mathematician, and software developer who has been instrumental to the development of Modal Interval Arithmetic and served six years as a committee member of the IEEE 1788 Standard for Interval Arithmetic.
In addition to his executive leadership at Modal, he is Co-founder and board member of RISC AI, Inc.
So, Anthoula, Nate- welcome to Re-think Health.
Anthoula Lazaris
Thank you, Maria, for having us as well and giving us the ability to present our collaborative project.
Maria Palombini
Oh, this is very exciting. I’m so interested to get to the nuts and bolts of this interview.
Okay, so I’m going to start with you, Nate. Maybe you could share a little bit with us what is a ALIX, A-L-I-X and what is the fundamental difference between a third wave versus a second wave AI tool? I mean, we’re just getting onto AI and you’re talking third wave, so obviously you’re already light years in front of us.
Nathan Hayes
No, that’s a great question and maybe to provide a little context here, I’ll even rewind and go back to the beginning and give an overview of where things started in the first wave back in the 1970s to 1990s, kind of roughly is the time period of what we would call the first wave of AI. These early AI systems were very good at reasoning. You know, playing chess or checkers, for example, but they didn’t really have an ability to learn because the way that they were developed is typically humans would program these systems with a set of rules, like what are the rules for chess, for example. And then the computer could use those rules to reason about the chessboard and act as an artificial opponent, for example, in the game. Things evolved roughly in the timeframe of about the turn of the century here, 2000 to present, I think, most people would characterize or agree that we’re still primarily in the second wave of AI and the main distinction here between the first wave is that in the second wave, the machines have actually become good at learning. So they not only can reason, but they can actually learn how to do something.
Machine learning, for example, is looking at a pile of photographs and saying, is it a cat or is it a dog. The machine by analyzing a large test or a training dataset of images, it can actually learn how to interpret the images and then after the training is complete, you can put in new images that the computer wasn’t aware of or that it didn’t get the seed during the training time, and it will then predict. It’ll say, oh, I think this is a cat or I think this is a dog.
So fundamental to this concept is you’ve got a training process for the second wave machine learning or artificial intelligence and in that training process, you’re analyzing very large sets of data so that the machine can find patterns in the data and it can learn. And then after the training process is finished, then you have deployment out into the field and the machine will then, what we call inference, or make predictions based on the results of the training process.
And from a mathematical perspective, what’s really going on here is this learning process is a very complicated non-linear global optimization problem. So that is the main characteristic of how these machines learn under the hood in a mathematical perspective. And the other characteristic that I think really defines the second wave that we’re currently in is that when the current algorithms and the current computers and methods that are used to solve this optimization problem are primarily statistical in nature.
The reason this is important to understand is that since everything is statistical, and confidence can only be measured for example, in terms of probabilities, you’re never really completely sure exactly where you stand in terms of how well of a job you’ve done with the machine. And in that sense, a lot of people have talked about using these second wave tools that it’s like working with a black box.
So as we talk about entering the third wave, the primary difference here from the second wave AI is that in the third wave, the machines become excellent at learning. And in addition to that, the machines begin to provide explanations. We’re overcoming that black box capability and we’re providing a more clear and concise and intuitive answers to the humans that are trying to work with the AI in terms of understanding how the machine goes about making certain decisions or predictions.
So this is what is very broadly called explainable AI since it’s kind of a new concept, there’s really a lot of different definitions and a lot of different groups that are starting to work in the third wave may have different definitions of what explainable means, but explainable AI from our perspective means that because of this new approach that we are using with the ALIX training method, which is built on this Modal Interval Arithmetic and it’s a completely different algorithm or method, if you will, than anything else that’s currently out there. The thing that is different is it’s not a statistical approach to training or solving that optimization problem. And so in that regard, we’re getting rid of all of these probabilities. We’re providing guarantees and repeatable results and answers through this process and through this unique capability, we’re also opening up that black box and providing a guaranteed view or answer to how did the machine, for example, arrive at this particular conclusion in terms of making a prediction that a picture contains a cat versus a dog or if in terms of healthcare, what we’re talking about today, is a patient healthy or they have a particular diseases.
Maria Palombini
Great, Nate. Thank you. Anthoula. Obviously Nate set the foundation for us on the technology. You know, we talk to a lot of clinicians and researchers and sometimes they’re like, oh, I don’t know about this AI thing when we’re talking about research. Can you give us a little insight about the case study, what you were going for in your research, and then at the end of the day, why you chose to move forward with a cutting edge AI tool, such as ALIX for this precision oncology research.
Anthoula Lazaris
When we talk about precision oncology, we’re talking about not treating just the disease, but treating the patient who has the disease. So really identifying unique features within that patient’s cancer. As we identify these unique features in the cancer, new technologies have evolved. For example, liquid biopsies, we hear about liquid biopsies.
This is what we’re doing here is we no longer need a sample of the tissue from the patient, which is very invasive. Instead we’re using a liquid form in terms of it could be blood, urine, saliva are just three examples. So with respect to the project that we have with Nate and where we started it. So with a basis, looking at precision oncology, really trying to focus on individual patient care and applying liquid biology, which is really in our case, looking at components within the blood that are either shed by or changed by the cancer.
The work that we do in our lab is focusing on colorectal cancer liver metastasis. We looked at the tissue and we identified markers that could predict a patient’s response to treatment, but we literally need tissue for this, which is not always practical when it comes to getting biopsies from patients. So the starting point of this project is we already had some predefined specific features within the tissue that we now said, well, let’s go into a liquid biopsy and see if we can identify these features in the blood and in essence, identify which patients will respond to treatment and which patients will not respond to treatment. And for this in the blood specifically, you hear a lot about circulating tumor DNA, where they’re looking at genetics. We took a different approach. We’re looking at these vesicles that are secreted by multiple different cell types and we looked at the proteins within these vesicles. So the starting point, the large amount of data we collected was vast amount of proteins from mass spectrometry data on the blood of two different populations of patients, those that do respond to current treatment and those that did not respond.
When we first met Nate, which actually was brought to our team from our business development office. So, as you can see, there’s a lot of multidisciplinary going on here. He presented ALIX to our team and we were really surprised that this type of analysis program, you call it third wave, actually existed. And at the time, Nate just referred to the basic bioinformatics tools, which really rely on statistical significance.
That’s a key feature here, I believe, because when we talk about statistical significance, so we pulled out based on our tissue and even looking at the blood proteins, we use bioinformatic tools on all the proteins we’ve pulled out of the blood. And we found over 50 proteins that looked like they were different between the two patient populations. But we had no idea which ones were important, which ones were not important. We couldn’t rank them to identify. So we’re screening now looking at 50 different proteins, which is very time consuming. So we were intrigued that ALIX could actually develop a signature for us. And also rank the signature and the biomarker found in the blood according to importance in answering our question, what will lead to a patient not responding to treatment versus a patient responding to treatment.
Maria Palombini
That’s fascinating. So much going on in the world of oncology research and to start to get at that level is critical, but really just amazing.
Anthoula Lazaris
First of all, we were surprised from the start in terms of what Nate and his team had developed in terms of, I wouldn’t even call it a software, I’d call it ALIX. So ALIX is our friend. The main outcome is we generated a signature that was able to tell us which patients would respond to disease, which patients will not respond to disease. And importantly, like I said, it was able to rank them as relevant and irrelevant.
The other thing that came to our attention was the way ALIX worked. So I’m a molecular cell biologist. I am not a mathematician or an AI person. What I had to understand from the beginning is that ALIX is driven by a multiplex analysis. We’re not looking here at identifying individual biomarkers. So it had to be clear from the beginning when we were first discussing with Nate and his team that we’re not looking at an individual biomarker, we’re not looking for a target for new drug here. That was not the goal of the project and we had to keep our focus like that.
Once we saw the signature, we said, okay, let’s apply our biological knowledge and look at different pathways and see what pathways are up or down regulated. It wasn’t that simple. Applying the biology to ALIX’s signature was novel.
It’s one thing to find a solution, i.e. the signature. It’s another thing to actually understand the solution. So we only had half the battle won at this point. So what we eventually through repetitive meetings and discussions with Nate, and I think that’s, what’s really important in this type of collaboration is Nate comes at it with his mathematical background and AI background. We were able to communicate, we’re able to understand each other’s languages. Whereas I was coming more from a biological sciences background, but through discussions, we were able to realize that ALIX’s solution was really telling us a whole body’s response to the disease. So it’s not just the tumor itself, tumor cells in the blood that people often find, et cetera. We’re not looking at that. What ALIX has identified for us is the body’s physiological response to the cancer. This is new. We had to figure out ways of trying to understand. So how do we now look at the whole body as a whole to understand what the signature means?
In essence, we had two major findings. One is we’ve developed a signature, which we will now move on to try to bring it into clinical practice. But again, that’s longterm still. And second is understanding what the solution ALIX is providing and how we could use that to better understand the physiology of the human body.
Maria Palombini
Wow, it’s unbelievable. I think that’s just amazing. I guess that’s when they say when you’re putting data to good work. One of the benefits of having both the technologist and the researcher on these kinds of interviews is that you can get both perspectives at the same time. So first I’ll start with you, Nate.
ALIX is scalable in performance and infrastructure like you mentioned, and is proven in software in this particular use case. But how can it successfully classify health versus disease patient and identify those biomarkers and those nuances that, Anthoula just came out and shared with us?
Nathan Hayes
Yeah, it’s a really good question and it goes back to what I was mentioning earlier in regards to, the training process versus the inferencing process. The McGill use case that we did with Anthoula, we analyze the data, using a method called K folds, which is basically where you take all of the data you have available and you basically partition it into K different folds where K could equal 5 or 10 or whatever number.
And the idea is that you set aside some of those folds or testing data and the rest of the data is used to train the system. And then after you’ve performed that training, then you set aside a different set of the folds for testing, and then you train again. And so this is a way of training the system, measuring capabilities in the field.
What we realized in this particular use case is that every time every single fold that we did, the training was always a hundred percent. And that was really important because therein lies the evidence of the hypothesis that, Anthoula and the researchers have that there really is a pattern in the data here that ALIX, because of the guarantees that it provides mathematically, based on the unique way that it finds solutions. It’s a proof performed inside the computer of the training solution. And so that is important to let the researchers know that they’re on the right path here, that there’s validation to their thought process. But in addition to that, the one other thing Anthoula mentioned is the ranking of the biomarkers and because of the Modal Interval Arithmetic method that we use with ALIX to solve the training as a by-product or as an outcome of those trainings with the ALIX software or method, we had a ranking of all the different proteins and, we analyzed thousands of proteins and out of all of those proteins, ALIX was able to rank them from the most important to the least important so that we could create a pie chart or a graph that we could provide with the researchers and actually identify by name what were the relative importance of all of these different proteins. And this again is all happening in a non-statistical manner. Basically, it’s a computational proof done inside the computer based on set theory that based on the data and the model that we created, this is the result.
Even though we still have work to do in terms of broaden the database of samples to improve the overall test accuracy of ALIX out in the field and we believe that’s going to improve with time. One of the things that we demonstrated with the K folds testing is that the ranking of the biomarkers did not change hardly at all, between all of the different folds. And so in that sense, you have a high degree of confidence that this list of biomarkers or that signature that Anthoula was talking about is not going to change even as the size of the training database grows over time.
Maria Palombini
It’s just amazing what this technology can do.
Anthoula Lazaris
You can just add to that. So the ranking in science is I can’t stress how important that is, but, ALIX also identified irrelevant protein markers. So you figured, okay. That’s the garbage, it’s not because when we talk about validation, like in a trial and you’re going across multiple different sites, different countries- how do you normalize your data? And that is a major issue in any type of clinical tool you’re going to develop, is normalization. So we haven’t yet finalized this, but we’re exploring with Nate, these irrelevant proteins that do not change between our patient samples. Could we use those to normalize data across sites?
So there’s a plethora of information that we’re still trying to understand in ALIX’s solution.
Maria Palombini
That’s amazing. I think, next time, if ALIX can talk, we should invite him to come to the conversation as well.
All right. I tend to do this to my guests. I’d like them to think quick and have a short, quick answer. So we’ll do this one at a time. So Anthoula, I’ll start with you. When I use the term, or I say the words, “AI for Good Medicine,” what’s the first thing that comes to mind and why?
Anthoula Lazaris
For me, good medicine, just first of all, is meaning improve patient care. So AI for improved patient care to mean means tools or technologies that support patient care.
Maria Palombini
That’s how I envision it as well. Nate, how about you?
Nathan Hayes
For me, I come at it from a little bit different of a perspective and that’s due mainly to my background as the technologist and the mathematician. But to me, the one word is ethics and using the AI in a responsible manner.
Maria Palombini
Absolutely. That’s leading into my next question for Anthoula. You touched on a little bit on this term validation but we often hear about ethics in AI and machine learning for healthcare, and it’s being used in multiple different ways and things, but given your experience now with this particular use case and having to use the application and seeing some of the outcomes and opportunities with it, what would you like to share with the global healthcare community about using these kinds of tools like AI or machine learning that perhaps they may not be aware or even misled when it comes to potentially having real impact in improving patient outcomes?
Anthoula Lazaris
When we look at the ethics component, there’s two things.
There’s data from the patient protecting the patient’s data. And there’s also ethical bias in terms of different patient populations. If we look at data protection in order for us to do the work that we just talked about today with Nate is on our side, on the hospital side. And on the resource side, we had to have ethics protocol to collect the data, but our ethics board and our protocols are very clear.
Any information I provide Nate or I put into any bioinformatics or AI software or technology, they cannot contain any identifiers. These are very well-defined in the ethics community- a date of birth, a day of surgery, names, of course, by far are completely out of this. And all of our data is actually double-coated.
You may ask why don’t you just anonymize? If you want to follow up on these patients to see if you find something interesting. If they’re anonymized, that means you can never go back to follow up on these patients. If they’re double-coated though, and this comes down to another ethics issue is if they’re double-coated and you identify for example, a disease that was maybe a susceptibility to Parkinson’s, et cetera, there are in your ethics protocol and in the consent of this patient there’s procedures put in place that you can actually go back to the patient’s doctor and let the doctor make that decision. That’s just a small example of one of the components that’s embedded in ethics. Our ethics in Canada, I can speak for, and specifically in Quebec, Quebec is actually more restrictive than the rest of Canada. It really protects the patient’s information and I think the patients need to be aware of that, but we can’t overprotect and not be able to go back to the patient to provide their doctors valuable information either. So we have to be aware that we still need to have that openness to go back to the patient when we need to.
With respect to bias, we work in colorectal cancer liver mats, by far it used to always be more male dominating in the older age groups. Unfortunately now with an increase in obesity, we’re seeing a shift in the younger population, but when we do select our patients like you do for a clinical trial, you are biasing your study based on who you know will benefit.
But I think what’s important is like you do in a clinical trial, you need to very, well-define your patient cohorts, the data you’re putting into it. So you already know that it’s going to be biased in what the bias implies. From my perspective, that’s the main two ethical issues.
Maria Palombini
Yes, very important. In blockchain, the quality of what you put in is the quality of what you’re going to get out. It’s almost the same concept and I think it’s really important.
We talk to technologists, they all have a whole array of things that comes to mind when it comes to a challenging aspect or gap that they’re finding in really driving the trust, the adoption, the mainstream acceptance, whatever you want to call it, you know, for the use of the technology in these applications. I guess my question to you is if you had to think of the single most challenging one that’s currently maybe not addressed in current discussions around AI or maybe just keeps getting pushed to the side, creating that little bit of uncertainty on credibility or trust in the tools, what would it be? And in your opinion, what may be one of the best ways to try to resolve it?
Nathan Hayes
Very good question. From a technology perspective, I think the main issue there is about leaving this paradigm of statistical probability behind into the third wave with ALIX and the guaranteed outcomes. But I think more broadly, even in a non-technical manner, I think the most important issue, there is something that Anthoula already touched on a little bit earlier, and that is the interdisciplinary nature that’s required for these programs and I think successful outcomes.
In my own personal view, this is one of the reasons our collaboration with McGill has been so successful is because of the way that our teams have worked together. Bringing our respective areas of domain expertise to the table through dialogue and discussion, being able to overcome the language barriers, so that we can really understand where each other’s coming from.
So we can really understand the medical hypothesis so that we can translate that into a machine learning hypothesis. So that we can take the machine learning results and translate that back into the domain of the medicine and the healthcare. It seems obvious, but the reason I point this out and answer it is because we do actually run into a lot of other scenarios, use cases, people just throw the data over the wall, kind of mentality.
And I think some of that’s just because these domains of technology and medicine are so far apart, it can be a daunting task to overcome that gap. But I think that there’s a lot of that going on. I get worried and concerned about that sometimes in terms of how is that really affecting the work and the quality of the results that are being arrived at using these techniques or methods?
Maria Palombini
Definitely something to think about. You both have shared such tremendous insight today. Any final thoughts that you would like to share with our audience, a call to action or something to get involved or take the extra step, whatever it might be in this pursuit of using these types of technologies to really start making an important impact in the area of precision oncology and research and that kind of thing?
Anthoula Lazaris
First and foremost communication, communication, communication. Like Nate just mentioned being able to understand each other’s language when you don’t know something, say you don’t know it and bring in others to help support you. I think that’s one key thing. And like Nate said, I think that’s why we’ve succeeded in what we’re doing so far.
And a quote. I can’t remember who said it, but basically it’s not enough to just do our best, but we need to know what to work on. With this specific example, we had one question, one hypothesis, and we got a solution. I find in science sometimes people are over-ambitious. They say, wow, ALIX is amazing.
They’ll try to feed it a whole bunch of data, but you need to stay focused and you need to have a simple question. Like Maria, you said at the beginning, we want pragmatic to be pragmatic. We want to be able to allow our patients to receive these solutions. In order to be pragmatic, we need to ask simple questions.
Maria Palombini
Very good point. And Nate, how about you?
Nathan Hayes
I would really like to just follow on that and add my second to it. It’s just so important to emphasize. I really do believe it is the most important thing to end on here that as exciting as all of these technologies are particularly ALIX and the new capabilities that it brings to the table, the machine learning and the AI, it is still just a tool. Everything in terms of the quality of the outcomes, the ethical nature really depends on the humans that are using the technology and how they work together.
Maria Palombini
That’s fascinating and very good parting points for our audience.
Many of the concepts that we’ve talked about today with Anthoula and Nate are currently being addressed in various activities here, IEEE SA Healthcare and Life Science practice. We cover a lot of areas of blockchain, AI, quantum, forward-thinking in mobile healthcare, telemedicine, whatever it takes to improve the patient outcomes across the healthcare value chain.
So we will include the links to Modal Technology Corp and the Research Institute at McGill University on the blog posts that’ll be accompanying this podcast. You can learn more about these respective organizations and the great work they’re doing.
Please check out the Healthcare and Life Science practice website at ieeesa.io/hls. We’ll have all the information about the different incubator programs we’re doing. They’re open for everyone to participate and to help us contribute towards global solutions to try to drive responsible and validated adoption of these technologies. I ask all of you to please, if you liked this podcast, please share it on your networks and actually use hashtag #IEEEHLS, or you could tag me Maria Palombini or the IEEE Standards Association. So we can give everyone access to this great information and this awesome case study. We want to get it out there and make everybody aware of what’s going on. I want to say a special thank you to Nate and Anthoula for joining us today. Nate and Anthoula, thank you. This was so great.
Nathan Hayes
Thank you.
Anthoula Lazaris
You also for having us.
Maria Palombini
Pleasure. And to all of you in the audience. Thank you for joining us. I want to continue to wish you to stay safe and well, and please keep tuning in as we bring the bright minds, such as the ones we’ve had today to keep sharing these great insights with me and with all of you. Until then take care.
Episode 3
AI: The New Pipeline for Targeted Drug Discovery
RNA splicing is at the forefront of providing insights into diseases that are linked back to RNA errors. Dr. Maria Luisa Pineda, CEO & Co-Founder at Envisagenics, explains how AI, HPC (high-performance computing), and genetic data can provide the insights needed for targeted drug discovery in oncology and other genetic conditions faster and more accurately than ever.
Speaker
Dr. Maria Luisa Pineda
CEO & Co-Founder, Envisagenics; Secretary, Alliance for Artificial Intelligence in Healthcare (AAIH)
Maria Luisa Pineda, Ph.D., is the Co-founder and CEO of Envisagenics. Dr. Pineda has over a decade of experience as a researcher and, before starting Envisagenics, she was a life science venture capital investor. Under her leadership, Envisagenics has received non-dilutive SBIR grants from the National Institutes of Health, generated significant revenue from Biopharma, raised capital from investors like Microsoft’s VC arm (M12), and won several prestigious artificial intelligence competitions, including the JLABS Artificial Intelligence for Drug Discovery QuickFire Challenge. To date, Dr. Pineda has secured research collaborations with Biogen and the Lung Cancer Initiative at Johnson & Johnson. She looks forward to closing more commercial partnerships in the near future to accelerate drug development with the help of SpliceCore®, Envisagenics’ AI platform that develops novel therapeutics for RNA splicing variants.
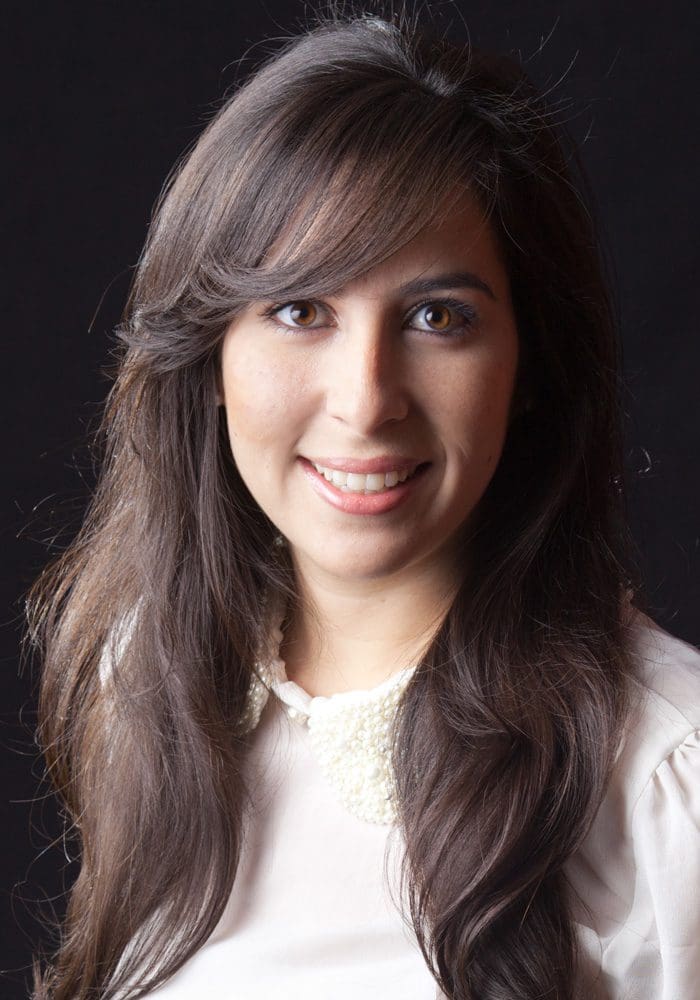
Full Transcript
Maria Palombini
Hi, everyone. Welcome to the IEEE SA Re-Think Health Podcast Series. I’m your host Maria Palombini, Director of IEEE SA Healthcare and Life Sciences Global Practice. This podcast takes industry stakeholders, technologists, researchers, clinicians, regulators, and more from around the globe to task.
How can we rethink the approach to healthcare with the responsible use of new technologies and applications that can afford more security, protection, and sustainable equitable access to quality care for all individuals? You can check out our previous seasons on ieeesa.io/healthpodcast or use your favorite player- Podbean, Apple Podcasts, Spotify, and more.
Here we are with season three: AI for Good Medicine, which brings a suite of multidisciplinary experts from around the globe to provide insight as to how do we envision artificial intelligence (AI), machine learning, or any other deep learning technology, delivering good medicine for all? We all want good medicine, but at what price? Essentially, in terms of trust and validation in its use.
As healthcare industry stakeholders, we’re not looking for the next frontier of medicine if it’s not pragmatic, responsible, and could be equitably valuable to all. In this season, we go directly to the technologists, clinicians, ethicists, regulators, and researchers about how these deep learning technologies can make real impact on improving outcomes for patients anywhere from drug development to healthcare delivery. Will AI, ML, or deep learning cut through the health data swamp for better health outcomes? Let’s find out. So a short disclaimer before we begin. IEEE does not endorse or financially support any of the products or services affiliated and/or discussed by our guest experts in this series.
It is my distinct pleasure to welcome Dr. Maria Luisa Pineda, Co-founder and CEO of Envisagenics. Welcome, Maria!
Dr. Maria Luisa Pineda
Oh, thanks for having me, Maria.
Maria Palombini
I am super excited with this interview. We’re going to talk about how we can get a better understanding of how artificial intelligence and HPC, high-performance computing, mixed with RNA sequencing is accelerating drug
discovery. So the mission of Envisagenics is to discover therapeutic points of intervention, to cure diseases caused by RNA splicing errors, using AI and HPC. Envisagenics partners with renowned institutions, such as Memorial Sloan Kettering Cancer Center, and has been recipient of grant funding from National Institute of Health and other world-recognized endowments.
I like to humanize the experience for our listeners. I’m going to start with a very important quote, and there’s a reason why I’m going to share this quote with everyone. “Behind every successful woman is a tribe of other successful women who have her back.”
I had the unique pleasure of meeting Dr. Pineda a few years ago at the Health Conference that was taking place in Las Vegas, just before the COVID pandemic broke out in the United States. I actually contacted her out of the blue through LinkedIn and told her I was hosting a session on AI and Women in Health and she immediately responded and agreed to speak in the session. From the first minute I met her, you can sense her enthusiasm and passion. Her dedication to inspire and share her story to mentor women in the field automatically made me think of words I have heard often from women like Robin Roberts, Sheryl Sandberg, and others when they say behind the successful woman, there’s more often a woman mentor behind her. Plus Maria being from New York, automatically I kind of right away just felt alive with her having that background. I felt like I was talking to one of my friends.
What inspired me the most when I was talking with you, Dr. Pineda is when you first opened my eyes, that AI will potentially become the new pipeline for drug discovery. She shared her work at the time with some unique findings on genetics of patients with triple-negative breast cancer. Their work exposed why these patients were not responding to chemo like in traditional therapeutic applications. As the day went on, she also randomly mentioned to me, she was hiring staff for her company and she said her preference was not to see the person’s name on the resume— I never forgot that. She said her interest was to see the qualifications of the person. Gender, race, ethnicity, or any other demographic indicator had no position in her decision for the right candidate. Like I said, I was inspired by the moment I met you in that meeting room. So I am so delighted to have you here today.
Dr. Maria Luisa Pineda
Aw, thanks, Maria. That’s really nice. You have great memory and it’s pretty impressive. So I’m very excited to be here.
Maria Palombini
Thank you so much. Tell us a little bit about you. You studied to be a biologist and had early success by being awarded an endowment of $2 million from the Goizueta Foundation. I’m not sure if I’m saying that, right?
Dr. Maria Luisa Pineda
Goizueta, yes.
Maria Palombini
And you were an NIH fellow and more. What drives your passion to not only help patients but also at the same time, your passion simultaneously to mentor women in the field?
Dr. Maria Luisa Pineda
Well, I was raised by a really strong woman— my mom— entrepreneur, businesswoman, raised three children by herself. So I think it starts by that— being raised by a strong woman and businesswoman. I learned and saw that. Now that I’m a mom, it’s even more important because I see how difficult it is, but how important it is and I could really see how women can do everything in their power and that they put their mind into.
What really makes me passionate always since I was very little, has been science. I’ve been doing science since I can remember and when I moved to this country, I ended up getting my mentor at Barry University and she was a German Scientist, a woman. Not only was she a great mentor, but an ally. She was always helping me on the science and I was a high school student back then and she helped me put a science project together and then I ended up winning and placing in the Intel International Science and Engineering Fair. With the funds I got from winning, I bought my first car, but that also allowed me to get a full fellowship from the Goizueta Foundation, which was run by the widow from the CEO Founder of Coca-Cola, who was Cuban-American. She was looking for a Latin X student that they could fund and after I won the science fair, she saw me in the newspaper and was able to give me that endowment; which what I was able to go to private school with. On top of that, then I was able to get NIH grants and when I pursued my Ph.D., I was able to also get Beckman, and Hearst Foundation Fellowship, and allowed me to realize this country provides so many opportunities for people that are interested in are proactive and are passionate in what they’re doing.
And for me, it was science, but while I was finishing my Ph.D., I realized I was not only able to do very good science but also all throughout my career, I was able to get my own funding for school, for research, and started a couple of groups of what can you do with a Ph.D.? Not only for females but for all Ph.D. students in the tri-state area, New York, New Jersey, Connecticut. Because I realized I could get my own funding and I was very good at this business thing, but I wasn’t sure what it was or what it meant, but I was mentored then by venture capital angel group founded by women to fund only women in C-level positions in companies, it’s called Golden Seeds.
And it was really impressive because women that start companies are in C-level positions actually have higher returns for their investment. So my passion is not only science, but also making a difference and having references and getting mentored by women and really good men allies, but being for other women in the United States, being that person for them and having a reference for them, because it is possible. You just have to make it happen.
Maria Palombini
Absolutely. I think it’s an inspiring story. This is why I started with the quote with the tribal women behind the woman is because this always ends up that way. I mentioned a little bit about Envisagenics, what the vision is, but how did a biologist by training, marry the science to this cutting-edge platform, using AI and high-performance computing, to accelerate these valuable insights that you guys are now generating and finding?
Dr. Maria Luisa Pineda
When we were at the lab, my co-founder, Martin. We were lucky to be part of Adrian Krainer’s development of Spinraza. We were seeing everything that was being done at the lab. And we partnered with Ionis Pharmaceutical and Biogen for children with Spinal Muscular Atrophy, which is a genetic disorder where children’s muscles stop working.
My professor, Adrian, and his team were developing in partnership with these other pharma, this small RNA therapeutic that could fix this RNA error in children with SMA, Spinal Muscular Atrophy. It took them almost 12 years, but the children, that couldn’t move a single muscle are now walking, are sending Adrian like little trinkets and pictures of him. That means their muscles are working again. They’re smiling again. So I was like, wow, that’s amazing. I wanted to do what they did with Spinraza, but instead, do it for many other indications. So what we were doing because Harvard did the human genome
sequencing. We had availability of sequencing and back then, one whole human genome could be a terabyte or so. So the data was starting to get bigger and it was very expensive in the beginning to do them, but the sequencing was getting cheaper and there was all this new technology that we could use instead of doing it on-premise, right on the computer.
We had this thing called the cloud that’s starting to be developed at the same time as sequencing. So the premise was using cloud computing, hyper-performance computing, so we could automate and accelerate what we were doing on-premise, right on your computer, in the lab faster.
It used to take us four to six weeks to analyze one dataset. Now we can do a thousand patients in under two hours. The premise of data and the amount of technology was exponential. So how do we use tech and high-performance computing or any sequencing machine learning so we could actually extract all that information and use it for therapeutic development?
While we’re building the company, we wanted to make sure that after we extracted truly meaningful data, we also had a biologist team that could then validate everything that came out of the AI/ML platform. It was very hyped in big pharma, so we wanted to make sure that we had a proof of concept that we actually were able to validate the findings that the platform was showing. Because, as my co-founder says, “everything is in the pudding.” Meaning, you really have to showcase tangible things because data information, it’s very abstract for us to generate therapeutic for patients so they could get treated and have better outcomes in their life.
We really had to take all those findings and validate them. So we did that with a case study that you said in triple-negative breast cancer, which has a very high unmet need for women in the United States and Europe. We have around now 50,000 women and there’s nothing available. What we wanted to do is use our platform at least to identify the right targets, which is exactly what my platform does, is identify that target for the right patient population. So then you can stratify them then design an RNA therapeutic or other types of therapeutic modality so we can target it the right way, finding the right drug for the right patients, basically. And then repeating the process over and over again. Machine learning and AI allow us to say no in a faster way, or keep putting more resources because what we do is extremely expensive.
In order for us, to accelerate and change the way that we do discovery instead of testing random drugs and see which one works, we actually identify the target and then develop the chemistry for that target, which then we can stratify the patients for that specific target, which becomes a component diagnostic as well as a therapeutic. We can do that now in less than eight months.
Maria Palombini
That’s really great. I think this is when we really think about the real potential of these kinds of platforms. But for some of our audience, who are not really scientists, they’re more on the technology side. So let’s just say we have to give a little bit more about this RNA thing.
We’ve heard about the mRNA vaccine from Moderna and Pfizer. Can you share with our audience what it means exactly by RNA splicing and the types of diseases that are most likely to be caused by this type of “error.”
Dr. Maria Luisa Pineda
First off, we’re heavily a health company based on RNA, so we’re very grateful that mRNA vaccines were brought to attention, but generally speaking, in order to create and make your body work properly, you have to create proteins. Protein is what makes all our tissues/our body function properly, but then, we all have the same genes. So, how do we all look different? That’s called RNA splicing. RNA splicing is how you cut and paste all those genes to make different proteins. That’s what makes all of us diverse. You look different than I do and even my siblings look different, but we all have the same genetic information. RNA splicing creates the diversity of the human genome and the proteins are being made. Then there’s many errors or some that are cis-acting or trans-acting. The cis-acting are like genetic disorders, just like spinal muscular atrophy, which is, making an error on one gene. Where in trans-acting, it’s more of when this splicing factory, which is the largest factory of yourself, has around 300 proteins. It tells you how the proteins are being built and when it gets messed up because it has 300 proteins, many things can go wrong. That’s where machine learning and platforms like mine could use machine learning and AI to basically look at opportunities and understanding how these errors are being made.
Most of the diseases that happens to are things like cancer- a number of solid or hematopoietic cancers, meaning, breast cancer, lung cancer, leukemia. Those don’t have a lot of DNA errors, but they have tons of RNA errors. When
you’re trying to develop a therapeutic, you should understand how that disease is being toxic to your body.
We use our platform to basically understand sequencing, and all these machine learning modules or algorithms to understand and extract all that insight of how the disease has been affected- which proteins are present in the disease patient and not in normal tissue. So when we were deciding the therapeutic, we’re hitting the right protein. Then if it’s too toxic, then we take it away, we put a bandaid (which is an RNA therapeutic), or we change it, or maybe use your immune system, which is fighting to make you feel better. We use the immune system to kill it, to go and eat it. There are different ways that RNA splicing errors could help.
I think the other ones are neuromuscular disorders or neurogenetic disorders. Those have 10 proteins that are messed up in most of those disorders. 8 out of 10 are RNA splicing factors. You really want to make sure that you can group patients by their RNA errors and understand what’s happening and the biology behind it. So then when you’re deciding drugs and therapeutics for them, you are doing it, understanding the mechanism of action and understanding what’s happening within the disease.
Maria Palombini
That’s just fascinating. I mean, just the insights alone. You’ve really hit on what the platform SpliceCore can do. What makes this platform so unique?
Dr. Maria Luisa Pineda
SpliceCore Platform utilizes RNA sequencing datasets for discovery purposes. For those that don’t know, once you have a tumor, that tumor normally goes to pathology so they can see what’s going on in the tumor. So you can get what type of cancer you have, what stage it is, but you also get a lot of sequencing, RNA specifically. We upload it to the cloud and then we use that against our transcript of reference like a map. We have approximately 7 million splicing targets. Instead of looking at genes, we actually don’t care about genes, we care about exons- three exons together, because they could be targeted by therapeutics.
By reenvisioning the human genome, and instead of calling these genes, we call these 3 exons and RNA splicing events, we now have 7 million of those. If you’re looking for something to drug and the database is only 33,000 then if you go through all your 33,000 and you do not find anything, nothing of your
chemistry set, then you give up. But when you have a 7 million target list, then you have more possibilities. Out of the 7 million, again, not all of them are good drugs and that’s where we use machine learning and AI.
We use different features because of having coincidences between data sets. Let’s say we find some samples- datasets from patients in John Hopkins Medical School, and then we go to the Broad Institute and then we go to MGH at Harvard and get three different datasets from breast cancer patients. And we find this target that is present in all three different cohorts of patients and not present in normal tissue.
Then we say, okay, this isn’t coincidence. Something might be going there. So we use these features, or I call them filters, and we diversify our approach instead of taking a funnel approach per target selection. Because in a single funnel, if we use the same filter for everything, once one goes bad, all of them will, because you use the same filter, right, or same approach? Instead, we use a diversified approach where we use different filters. By doing that, then we can identify targets for different modalities. Modalities is the type of chemistry that you use for drugs. So you can have targets for RNA therapeutic or antibodies or for small molecules. Depending on the chemistry that I use, the targets will look different, so the proteins will look different. We really use the platform like that.
Maria Palombini
That’s just awesome. These levels of data that your guys are getting to is just unbelievable. How have you managed to keep it secure, private, compliant? I’m sure you guys have all of these challenges having to do that.
Dr. Maria Luisa Pineda
Data is a core piece of our company, Envisagenics. I think we’re very fortunate because we have Microsoft as one of our partners. I think Microsoft and other cloud service providers have done really well for us to have built our platforms with them, or while they were starting, they basically were really worked on
security and privacy and compliance. We frequently interact with the engineers and knowledgeable experts so we can assist with the growth.
But in reality, we focus on the science and building our platform and evaluating things in our lab while companies like Microsoft, AWS, and Google- all three of them have really focused on securing things on the cloud and having everything very compliant.
But, all the data that we analyze is de-identified. We have absolutely no data that could be tracked back. And even because of that, the platform is almost double de-identified because once it goes through our platform, we have different types of files that come out of that. Once it comes out of the specific SpliceCore outputs, those outputs cannot be back engineered and can only be analyzed or read by SpliceCore. That could be also put into different cloud service providers or partners. We can add it to people’s tech hub, or cloud, and if we need to analyze their data, we’re not moving it. When you have patient data, you don’t want to move it, store it or do anything with it for privacy. So what we do is that we bring our platform to that dataset. Our specific file outputs I was telling you about. Those data outputs is what we use for drug discovery and development. They don’t have to touch, move, or, do anything with anybody’s data.
We took that approach on the technical. It was a big bottleneck in the beginning, but working very closely with Microsoft and other cloud service providers, helped us to build our platform in the cloud with HPC, from the get-go. That has allowed us to focus on the science and leave all that data management for them.
Maria Palombini
That’s awesome. I hear this a lot now, too, with swarm AI and decentralized research, and it’s all about sharing of the insights, not so much the data.
Dr. Maria Luisa Pineda
There’s so much data out there, it’s what you do with the data that makes a difference.
Maria Palombini
Absolutely. So you’ve already shared some interesting outcomes from your work. What do you think are some of the greatest contributions you guys have had either towards the development of a targeted therapy or, the total amount of reduction of time in finding out particular insights that were not there before, or all of the above. What do you think has been some of your greatest contributions so far?
Dr. Maria Luisa Pineda
I’ve mentioned the triple-negative breast cancer using our SpliceCore platform and the downstream validations that come with it. But basically, we went from
data analysis of RNA sequencing all the way to a novel preclinic called Target and its compound in a matter of eight months.
So our platform can predict this optimal binding for RNA therapeutics. Our machine learning algorithm predicted five and two of them worked. To this date, scientists for RNA therapeutics, do something called microwalk where they go in one base at a time. So they manually test over 200 nucleotides upstream and downstream and two to three work. So imagine we’ve predicted five and synthesized five and two of those worked because we understand all the biology behind it. Each of those compounds is worth $3,000 to $5,000 to just synthesize it and a timeline to get it done in a matter of months or years and we just cut the cost so you can test a small fraction without having to waste all those resources on time.
That’s one of the things that deep learning and AI platforms like ours could definitely, you could see it right away, right? Just by the numbers.
Maria Palombini
That’s cool. That is really fascinating. Definitely helps expedite nearing that race, anything helps.
I hear this debate. There’s no such thing as precision medicine, or we have to move towards precision medicine, but I noticed something specifically when you talk about targeted drug discovery, you use these words very selectively. So I kind of want to get your input as like, what do you think is the difference and why is it better to more accurately look at therapeutics through that lens?
Dr. Maria Luisa Pineda
I think precision medicine is not very specific per se, where was targeted drug discovery. We at Envisagenics believe that if you find the right target for the specific indication or specific disease, then you understand you can stratify patients so it’s finding the right therapy for the right patients. That’s why we call it targeted because we find the target, we understand the mechanism of action, the biology behind it, and try to understand the disease itself and how the target is involved in the disease. Then we designed the chemistry against that target. So once we’re going into the clinic, then we can stratify the patients. We can understand what error we’re fixing on that patient population. And I think it’s more accurate; it’s a better term. It is the future of medicine.
We all have to be treated in a targeted way because cancer just means when the cell was bad. We all have the same genetic material, but there’s so many things that are involved in diseases, pathogenesis, right? If there’s so many things could go wrong, you really have to understand what’s going on wrong for each person. But if we could group those patients, then we can target them in groups instead of one by one. Precision medicine is one person and that takes a long time. But if you can group them, then we could target them by groups and stratify them and save more patients’ lives faster.
Maria Palombini
Absolutely. Very good point. I like to do this to all my guests. I call it the think fast question. So when I mention “AI for Good Medicine,” what is the first thing that comes to mind and why?
Dr. Maria Luisa Pineda
AI in biopharma has come so far. Eight years ago, let me just put it that way, we were starting to be invited to panels and there was the same five companies. Maybe 50 people in the room were talking and now, some of the AI panels that I’ve been, we have 2 to 5,000 people that are interested.
So the AI sector in biopharma industry has grown so much, and it’s such an essential part of this organization because we really have to use innovation to change. I mean, look at COVID the way that it happened, right? We use innovation, we use Biointech, they did such an amazing job, and everybody came together in a year to have a compound being tested in clinical trials, developing it, and all the pharma. AI was such an important part of this and it’s very promising. It will bring forward new therapeutics that we’re hoping to reach patients that are in big need and their families. So we can accelerate change and in a group effort really bring forward what has not been previously possible without traditional methods of just failing one by one.
Maria Palombini
Absolutely. One of my first podcasts for this season, we had a debate on whether the ethics of AI. It was all about, does it cause more healthcare disparity or actually close the gap? But in this case, with you, it’s more in the question of validating responsible use in AI for health applications. So you have the work in the science and now the tech side, what would you like the global healthcare community to know about these types of tools that perhaps they may not be aware or misled when it comes to potentially improving the patient’s health outcome?
Dr. Maria Luisa Pineda
I think AI, you really have to work together in order for you to use it the right way for the right means. For us, at least, is for drug discovery. So understanding from the patients what’s going on in developing therapeutics for them.
We created a global advocacy organization that is dedicated for the discovery and development and delivery of better solutions so we can improve patients’ lives. That is a coalition between technology developers, pharma companies,
research organizations like mine, universities and the US and European governments, and Canada. We put in together so we can realize the potential of AI and machine learning in healthcare.
How can we improve the quality of care? But addressing the industry challenges like publishing responsible, ethical, reasonable standards, how we’re developing policies, working with government, NGOs, key opinion leaders, and other international stakeholders. So we can have the premise or the promise of AI and how it works and how it can improve patient’s lives, but at the same time, making it efficient, sustainable, and creating an accessible healthcare system that is diverse. For instance, we all use data. We want to make sure that the data is coming from a diverse set of populations. We’re going through COVID, that happened, right? So, you know, minorities and underrepresented groups and women tend to not being part of those clinical trials so then we didn’t have a lot of data going in. So we always say, ” garbage in and garbage out.” So as data scientists and innovators in healthcare, we have to make sure that we have a wide variety of participants across the healthcare spectrum, as well as the diversity of data in patient population because diseases are global. They’re not one race. They’re affecting us anywhere in the world. That’s why you have to think about these issues globally, but make sure that they include us in the conversation. So when we’re standardizing, we were part of that as well as the government, pharma companies, and some of the academic institutions because they don’t have enough knowledge so combining everybody into one organization, like the Alliance for Artificial Intelligence in Healthcare, has allowed us to come together and set something up. So I want to make sure that everybody knows, I guess we’re working towards a healthier future, but all of us as a group. And I think that’s extremely important for our children and medicine in the future.
Maria Palombini
Absolutely. Really important to note that diseases don’t have a bias. You talked about so many great opportunities and insights. Talking to some of my guests, they always say that there was some single most challenging aspect when they
started or as they go through the process when they were using the applications of AI. It could have been lack of open data or there’s not enough standards developed, more policy, or it could be not enough computing power. And all of these things just seem to somehow fuel some concern or uncertainty or credibility and trust of these tools. So for you, what was it, or what could it be and why? And in your opinion, what would be the best way to resolve it?
Dr. Maria Luisa Pineda
So I’m the type of person that if it’s not there, you can create it. One of the co-founders of the AIH, or the Alliance of Artificial Intelligence in Healthcare, said if there’s no policies, then let’s get together. There’s no standards, let’s get together and figure them out, put them together with the government, with the regulatory institutions so we can get things done.
I was the vice chair for a year and a half and now I’m the secretary of the AIH. We really work together with my other AI in drug discovery or in healthcare companies and the pharma partners to really work on all of these challenges that we all face. It’s not only Envisagenics, all of us face the same thing. So grouping it and working together will help us apply it and resolve it. And then when it comes to, all the technology or a cloud or bottlenecks. Once you have a bottleneck, then you go and work with people that could help you solve it either government or providers.
But again, you need to have that proactiveness and that vision and be willing to work. Just because there’s challenges doesn’t mean that you shouldn’t do it actually it’s the opposite. If there’s challenges, they need to be resolved. If you don’t try to resolve them then who will. So I’m always trying to put a foot forward and be part of the change and try to resolve things as best as we can and bring the opinion leaders and the leaders on each of those fields so we can resolve it together.
Maria Palombini
Absolutely. I think it’s a very important approach. You all sharing the same challenges that are maybe blocking innovation or really not letting you open the doors the way you want to. So the best thing is to get together and figure it out.
I am familiar with the organization and I’m all for the great work that you guys are doing over there.
Dr. Maria Luisa Pineda
I appreciate it. It’s been two years and a half that we’ve been building it from scratch, but it’s definitely necessary not only to work together but also help each other and partner with each other.
Maria Palombini
Maria, you’ve given so many insights and so many thoughts today. Any final thoughts you would like to share with our audience? A call to action for any technologist considering getting into this space or is already in that area of health tech, but not really sure where they’re going with it.
Dr. Maria Luisa Pineda
I really want to say that if it was easy, everybody will do it. Just because it’s hard doesn’t mean that you shouldn’t. And so I’m always saying, just go for it. Ask for help, get mentored, get allies, get partners.
And by doing that and having the right team in place, you really can accomplish anything you want in life while having a balance and balance means very different things for people. I’m a mom, a wife, I’m a CEO and I couldn’t do anything if I didn’t have all the support from my mentors, from my team, from my husband, from my son.
Having that balance, whatever that means to you, will take you places that you will never imagine that you could.
Maria Palombini
Absolutely, it takes a tribe. I think that’s really important. So for all of you out there and make sure you have the right support system. I think it’s really important to achieve your goals.
Maria, thank you so much. I want to thank you for your time, but especially for the great work and making yourself available to talk with me today, I greatly appreciate it.
Dr. Maria Luisa Pineda
Well, thanks so much for the invite. I always love talking about all the work that we’re doing, the amazing science that my team is building. And again, it’s all for
the patients and their families, so we can get drugs and therapies available for them as soon as we can.
Maria Palombini
Absolutely. So for all of you out there, if you want to learn more about Envisagenics visit Envisagenics dot com.
Many of the concepts we talked about today with Maria are addressed in various activities here at the IEEE SA Healthcare and Life Science Practice. The mission of our practice is engaging multidisciplinary stakeholders and have them openly collaborate, build consensus, and develop solutions in an open standardized means to support innovation. And that’ll enable privacy security and equitable, sustainable access to quality care for all.
We have activities such as WAMIII, Wearables and Medical IoT Interoperability Intelligence, Transforming the Telehealth Paradigm, Decentralized Clinical Trials, Responsible Innovation of AI for the Life Sciences and a whole bunch more. If you’d like to learn more about these activities, they’re all open. Meaning you can just join. You don’t have to be a member or pay anything. And you want to contribute your expertise in solving a major challenge to open the doors to innovation, please visit ieeesa.io/hls.
If you enjoy this podcast, we ask you to share it with your peers, your colleagues, on your social media. This is the only way we can get these important discussions out into the domain by you helping us to get the word out. You can tag us on Twitter @ieeesa or on LinkedIn, IEEE Standards Association when sharing this podcast.
I want to do a special thanks to all of you, our audience for listening, continue to stay safe and well until next time.
Episode 4
Reducing the Healthcare Gap with Explainable AI
Fairness is not a math problem. Healthcare disparities are a global challenge requiring more than just physical care. Identifying and leveraging social determinants, when mined correctly, are untapped keys to closing the healthcare gap.
Join Dave DeCaprio, Chief Technology Officer & Co-Founder at ClosedLoop.ai, and our host, Maria Palombini, as they discuss how off-the-shelf AI presents a new perspective on transparency, reduction of bias, and a path toward health stakeholders’ trust with explainability in its applications.
Speaker
Dave DeCaprio
Chief Technology Officer & Co-Founder, ClosedLoop.ai
With over 20 years of experience transitioning advanced technology from academic research labs into successful businesses, Dave cofounded ClosedLoop in 2017 to build a healthcare-specific data science and machine learning platform. ClosedLoop was selected the winner in the AI Health Outcomes Challenge, a $1.6 million X-prize style competition sponsored by the Center for Medicare and Medicaid Services, and was selected as a Top Performer in Healthcare-focused AI in 2020.
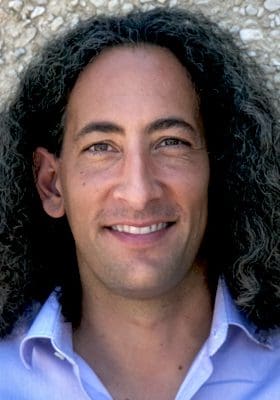
Full Transcript
Maria Palombini
Welcome to the IEEE SA Re-think Health Podcast Series. I’m your host Maria Palombini, Director of the IEEE SA Healthcare and Life Sciences global practice. This podcast takes industry stakeholders, technologists, researchers, clinicians, regulators, and more from around the globe to task. We ask them, how can we rethink the approach to healthcare with the responsible use of new technologies and applications that can afford more security, protection, sustainable, equitable access to quality care for all individuals? Yes, this is an ambitious goal, but a very important one.
We have previous seasons of our podcast series. You can find them on ieeesa.io/healthpodcast, or you can use your favorite podcast player- Apple, Podbean, Spotify, and more to find us. So here we are with Season 3, AI for Good Medicine, which brings a suite of multidisciplinary experts from around the globe to provide insights as to how do we envision artificial intelligence, machine learning, or any other deep learning technology, delivering good medicine for all.
We all want good medicine, but at what price? Essentially, in terms of trust and validation in its use. As healthcare industry stakeholders, we’re not looking for the next frontier of medicine, if it’s not pragmatic, responsible, and can be equitably valuable to all. In this season, we go directly to the technologists, the clinicians, and the researchers, the ethicists, and ask them about these deep learning technologies— can there be real, trusted impact on improving outcomes for patients anywhere from drug development to healthcare delivery. So here it is, will AI, ML, and deep learning cut through the health data swamp for better health outcomes?
So just a small disclaimer before we begin. IEEE does not endorse or financially support any of the products or services discussed in our podcast, at any time. We’re here to interview the experts based on their innovations and their experience in the field.
It is my pleasure to welcome Dave DeCaprio, Co-founder and CTO of ClosedLoop.ai, to our conversation. Welcome, Dave!
Dave DeCaprio
Thanks!
Maria Palombini
Great. So we’re getting into a conversation about a better understanding of explainable AI and how to better close the healthcare gap. ClosedLoop.ai lives by a mission to improve health and transform care with data science and AI. They are a winner of many different innovations awards, and most notably in 2021, they won the $1.6 million grant from the Centers for Medicare and Medicaid Services Artificial Intelligence Health Outcomes Challenge— one of the largest healthcare-focused AI challenges in history. They beat out some hefty competition and we’re going to get to it into the core of the interview.
I often hear that successful entrepreneurs are those who are passionate about the topic or the mission of their work. I read this story about your Co-founder, Andrew Eye, about his daughter. She was on the verge of a liver transplant after an autoimmune hepatitis diagnosis of her liver. Ultimately and thankfully, a prescription for prednisone saved her from that fate. But the moral of the story, Andrew later learned that in half of all pediatric liver failure cases, they’d never had a diagnosis and in 15% of those cases, they never ran the autoimmune hepatitis tests. No one had ever used past data to improve that clinical decision. Which is where ClosedLoop.ai is hoping to change that course. This is why I’m so excited to have this podcast with you, Dave. So Dave, tell us a little bit about you. I know you’re a Co-founder of ClosedLoop and knowing the story behind Andrew’s daughter, what drives your passion most in this work? How did you get here?
Dave DeCaprio
Yeah, I think part of the reason that story is so powerful is because it resonates with everybody. Everybody has some connection to the healthcare system and an example of where it hasn’t worked great for them.
I grew up watching my older brother struggle with rheumatoid arthritis before there were any effective treatments. And as a kid, I always knew there wasn’t much difference between him and I, but I was able to run and jump and do all kinds of things that he wasn’t able to. That just never really felt fair to me and I think that underlying unfairness, just because he happened to get a disease that I didn’t, I think has driven a lot of my passion for healthcare.
I’ve been in some form of AI in healthcare and life sciences for about 20 years now. I got started with the opportunity to work on the human genome project, the original sequencing of the human genome at MIT. And then was in drug discovery for a long time. One day I was working in drug discovery and I thought to myself, “I don’t think the problem with healthcare that’s most important is that we don’t have enough pills. There’s gotta be something else.” So I started looking around at what were the problems with healthcare and what were the ones that I, as a computer scientist, would have some ability to help fix and that’s how we ended up in the space of trying to figure out how to use all the available data we have to just make all the right decisions, using all the information today. There’s so much technology and treatment, and there’s so many therapies, but we’re not always giving the right treatment to the right person at the right time, given the data we have.
So that’s been my mission.
Maria Palombini
Absolutely. I think everybody has a healthcare story. I also have a similar situation— family members misdiagnosed with cancer, then it was caught too late and then we all know how it works in oncology when things go too late. Everybody has that similar story and it’s really inspiring that you guys take those challenges and turn them into hopefully a cure in some form or another.
What’s ClosedLoop’s, philosophy on tackling healthcare challenges and changes? What is the vision of bringing this innovative, “off-the-shelf” approach to AI tools, this commitment to transparency that you guys are all about?
Dave DeCaprio
As far as the philosophy on tackling healthcare challenges, I think, one of the most important driving factors for us is really humility. Healthcare has enormous problems. It’s super hard, and there are a ton of smart people working on it. And you can’t go in with an attitude of, “we’re going to have this magic algorithm that fixes healthcare, and then everything’s going to be great and we’re going to revolutionize the industry.” No matter what you do most of the smart people are working somewhere else. And so we’ve really tried to focus on, hey, what are practical things we can do to actually make improvements today with the technology that exists today? And importantly, we try to think about not just how we can build an algorithm that does something, but how do we build a set of tools that make everybody a little bit better at doing this?
There’s no way ClosedLoop is going to be able to solve all the problems, but maybe we can make some tools, that’ll help a much larger group of people be able to really make a bigger dent in the problems we face. If you start with that perspective, then you start to think about “how do we make what we do transparent?”
People can’t use our tools if they don’t understand them. How do we make them as simple and robust as possible? And sometimes that means not using the most advanced technology we can find, but trying to use the thing that’s going to work the most, the most often. And so that’s how we try to approach these problems.
Maria Palombini
I’m glad you mentioned that it’s a tool and it’s not going to solve everybody’s problem. But the idea is that everybody’s working towards contributing to solving the problem.
I briefly mentioned the awesome award that ClosedLoop won, but let me give a little background.
The challenge was focused on explainable artificial intelligence solutions to help frontline clinicians understand and trust AI-driven data feedback. We all know this is a massive concern in the industry. And it was to demonstrate how AI solutions could predict unplanned hospital admissions and adverse events, which is a $200 billion problem that impacts nearly 32% of Medicare beneficiaries. This information coming to us from CMS.
For those of you outside the United States, the CMS is the Center for Medicaid and Medicare Services. It’s a government-run payer for certain citizens of the United States, either driven by age or disability, or some other factor. So, Dave, you guys beat out some competition, some large multinational organizations.
Can you tell us what made ClosedLoop’s patient health forecast stand out among the competition? Where did it excel most to meet or exceed that tough judges panel expectations?
Dave DeCaprio
This is always a fun one to answer. There’s a lot that goes into winning a challenge like this. I think the most important thing is you have to believe you can. When we submitted the application, there were 300 plus teams: IBM, Watson, the Mayo Clinic, the Cleveland Clinic, participating.
It’s a lot of hard work and the first thing is you got to believe that you can actually win so you’re willing to put in all the effort it takes to win. Second, one of the things that I told the team throughout the contest was the overall quality of our submission, it’s going to be dictated by the dumbest mistake we make.
I think one of the things that really distinguished our solution was not having any weak spots. There were three parts of the contest: accuracy, explainability, and fairness. And we pushed really hard in all three of those areas and tried to make it so that every element of the solution reinforced every other element. And we didn’t have any spot where we felt like the solution was weak or we weren’t doing something that somebody else would have thought to do and could’ve seen. On the patient health forecast, in particular, this was a user interface to explain the predictions and help drive further clinical interventions.
As a software company, we approached it as a software user interface. It was a particularly important one, one that shows predictions of people’s future health, but the same qualities of user testing, user research, doing lots of incremental iterations. We did like 17 different iterations of that patient health forecast, every time getting more and more feedback on it. What did people like? What did they understand? What did they not understand? And it changed a ton from the beginning of what we thought would be valuable to what people actually found valuable. And so many things approaching it with just a discipline and a process and being willing to put in the work on all those iterations. I think ultimately made something that stood out against the competition.
Maria Palombini
We’re going to get now into the next part about exactly how this sort of project went on. But I think it’s just amazing, the approach.
So ClosedLoop is based in Austin, Texas. Unfortunately, in major Metro areas, we see there’s always a disproportionate rate of disease. Such as cancer, diabetes, and even COVID-19 amongst people of color. These are often marginalized communities that don’t have access to healthcare. So I know that Dr. Jim Walton, Presidency of Genesis Physician’s Group in Dallas, who reached out to you guys to help them sift through the social clinical data of 30,000 Medicaid patients to identify who would be the most risk of getting ill and would have the most significant outcomes as it relates to COVID-19. You get this request, right? You get this opportunity. What are some of the considerations when you first looked at this project and said, okay, we have all this data, how are we going to validate the findings?
I’m sure that you guys were like, wow, this is a great opportunity, but there’s a lot here to go through.
Dave DeCaprio
This was a fascinating project. I think one of the really interesting things about it is a consideration that happens nearly every project we have which is trying to make sure that you’re building an AI-based predictive model, but that it actually maps to some use in the real world in some actionable decision or intervention that can occur.
I’ll explain why that was particularly important in this case. First, there’s a lot when you approach a project like this that you have to understand about just good hygiene, essentially, in building a predictive model, making sure you’re doing appropriate historical backtests, you got representative populations. You’re checking that the model is performing well across all different groups. It’s not biased towards one or another. Those are a bunch of checks that you need to just understand and do and we consider those kinds of table stakes for operating in this space. The good thing is most of that stuff is well documented and if you just follow data science best practices, you can kind of get there.
Where this project got interesting, was that what was going to happen with these predictions? People who worked for Dr. Walton were social workers. This was not doctors that these predictions were going to. These were social workers who are going to be able to reach out and help people overcome some of the barriers they might have to treatment. Because these predictions were going to social workers, the kinds of interventions they could do were more around the social determinants of health than the clinical aspects of it. So these weren’t people who are going to be prescribing new drugs or ordering tests or giving treatments. But they were somebody who could arrange childcare or transportation or get somebody into a community-based program or enroll them with a community food bank. They were having problems getting meals.
So when we looked at what was available to those people, it turned out that what they really needed to know was who was the most likely to have these problematic outcomes? Where that outcome could be improved by addressing some social factors, something else that a social worker could get to.
And so when we built that model, we actually didn’t include every single piece of clinical information we had available, but we focused a lot on including all of the social determinants information so that when we gave those predictions back, each prediction came with some identification of what were the social determinants that were likely modifiable for this person that could actually improve it.
There’s a big difference between just predicting something and predicting something and saying, hey, here is something that you can actually go do with it. That’s one of the fascinating things in this project is it wasn’t about putting this thing in front of a doctor. It was about putting it from the social worker and seeing what they could do. And that actually affected the model that we built.
Maria Palombini
Wow, that’s a fascinating approach. Usually, you always think about the physical right? The clinical side of health, but there are so many social determinants that are barriers, right? To getting, like you said, they can’t afford, or they have childcare or whatever issues.
Another way of looking at the problem. And obviously, the data with it was probably just an awesome finding.
For our audience out there, the goal of the competition was not just about accuracy. When we think about AI, we’re always thinking about accuracy, of course. But it was about explainability and transparency. We all know with physicians with AI, they’re like, I’m not so sure about this thing and how is this all going to work?
What makes ClosedLoop’s software explainable to physicians who are not technologists but they need to use it for better clinical decision making. What exactly does it even mean to be explainable AI?
Dave DeCaprio
I can tell you what it means for us. I don’t want to get into a debate with anybody about what explainable AI means for everyone and what the official definition is, but I can tell you what it means for us. For us, that’s providing with every prediction that we make, the reasons why.
The system doesn’t just put out a number that says, I think you have a 92% risk of going to the hospital in the next six months. It says I think you have a 92% risk of going to the hospital and the baseline risk for somebody at your age with your overall conditions would be 65%. And that difference is because of the following specific things I’ve seen about you. And by “I’ve seen” I’m anthropomorphizing that the algorithm a little bit. But with each prediction comes with: hey, I’ve noticed you were at the emergency room visits recently. You were in the hospital. You’ve had an increase in utilization. Your drugs have changed recently. You had to change your prescriptions and that’s often associated with complications when somebody gets on a new drug. Or maybe you’ve stopped taking one of your medications, and we’ve seen that in your refill records. There are all these individual items that can come up that affect it. And when you show the prediction along with those reasons why that provides explainability. Now, what you don’t have to do is try to explain all the details of how the entire algorithm works and all the math behind it. Clinicians don’t generally care about that.
What they do care about is seeing here’s what the prediction is. Here’s what the baseline for somebody like that would look like and here’s the reasons why this person is a little bit different or here’s what special about them. If you can demonstrate that and show that those reasons make clinical sense to a clinician, that’s the way they gain trust and other clinicians. They talk to them about the decisions they make and why they made them.
And then those decisions make sense. So then they agree. You also need to have enough statistical rigor and enough scale that you can prove that the individual cases people are looking at are representative of everything. But really it’s about explaining an individual prediction. The math part of this is pretty much available.
We use a technique called SHAP Scores. There’s a couple other techniques available. The underlying math is pretty well laid out, but how you present that to a clinician is really important. How you figure out the right significance cutoff for what is important to show and what isn’t. How do you explain those things in normal English terms so that people can understand them?
That’s a lot of what we build around the underlying predictions to make these things actually comprehensible so that people can understand the individual predictions. The interesting thing about explainability is once you start using it, you realize how incredibly valuable it is for not just the explainability piece, but everything about what you’re doing.
When the models come up with a top factor and a top reason why that doesn’t make any sense, it’s just a great trigger that something has gone wrong in the data. Something is up with the validation of this model. If the model suddenly says that, oh, nobody has been taking their prescriptions for the past two months, well, maybe we’re not getting the right prescription data anymore. And we can see that kind of stuff. And it pops right out in the model.
That’s how we approach explainability. I think there’s other approaches that different people have made. And I think we’re all just trying to figure out what’s the right way to do it.
Maria Palombini
It makes sense. We always hear people say if you don’t understand something you tend not to trust it. I think that the explainability from that point of view, if a doctor can understand the points of reference, how it got there, then it all comes together. So I think that’s definitely an interesting and valuable approach, especially for overcoming that barrier of trust when it comes to physicians from that point.
Interestingly, trust. That comes up again, of course, with AI. We see that AI for healthcare tends to trend towards this proprietary algorithm, to solve whatever issue in the healthcare domain they’re designed for. But this sort of proprietary kind of seems to further fuel the distrust amongst physicians. We’re really concerned that these tools may not account for all the patients equally in their patient pool. So this question of bias and how these things arriving at these decisions.
My question to you is how does ClosedLoop mitigate those concerns for this potential bias by giving tools to health systems, to build their own algorithms? Obviously, with the understanding that they might have the tech team to support that kind of effort.
Dave DeCaprio
This is definitely something we see. We talked to several of the bigger companies and they always want to have this model store where you can come in and just pull algorithms off the shelf and then deploy them in your environment.
I couldn’t disagree more with that approach. Maybe at some point in the future, it might be feasible. But I think if you look at the state of the technology today and the state of the data today in modern healthcare organizations, we’re not at the point where you can make an algorithm or a model in one place and then apply it everywhere.
If you have a proprietary algorithm that works off a very fixed data set like an MRI machine that has a built-in algorithm to predict some aspect of maybe ejection fraction for a heart MRI. That can work very well because the data is constrained. But once you start looking at people’s wider medical records and longitudinal data and integrating many different data sources across healthcare organizations, what you end up with is that so much of the variability of the system is in each company’s individual data layout.
And so the idea that you could somehow take one algorithm and apply it in all those things, and be able to validate that at all, it doesn’t make any sense. You have to validate the actual system that’s running, which includes all of that data. And so our approach is very much to how do we very quickly go into an organization, take the data they have available, build and vet a model on their data. So we can actually get their historical data and do historical backtests and validation on their data with their population. And then explain how those models work so that the people who are involved get a sense that this is not just going to work in the abstract. They know that this is going to work on my population.
It is then possible to get that kind of trust. I don’t ever want to say it’s easy to get trust of clinicians and it shouldn’t be. It should be a high bar to get, but it should be possible if you can show them that it’s going to work in their entire system.
Maria Palombini
Absolutely. Physicians have to earn patients’ trust. So I think when we look at the chain, that’s pretty much the way it will go, for sure.
I like to ask this question of all my guests, think fast type question. So here it is. When I mention “AI for Good Medicine,” what’s the first thing that comes to mind and why?
Dave DeCaprio
I guess I’d say health disparities. The difference in healthcare outcomes, particularly in the US, based on a variety of factors— race, socioeconomic status, gender. There are massive disparities in the outcomes that people have that are not dictated by biology. They’re dictated by societal differences. And these are huge problems. You look at the problem of health disparities, socioeconomic status, nearly every model we build, if you include socioeconomic status as a factor, it comes up as significant. So it is always important. For nearly every outcome that we look at, this is a really big problem. It’s something where it requires an active fix. If you just use AI and machine learning to build systems and don’t think about actively reducing health disparities, what you’re going to do is embed those disparities in the systems that you build.
So it’s not a problem you can even ignore or deal with later because you will make it worse if you build systems today. There is a huge example of this with a model that was trying to select people for chronic care management programs based on their prior healthcare costs. There are racial differences in how much it costs to treat the same illness among different races.
And so that model ended up being racially biased because it was treating people who historically were more expensive, were being treated by the model as being historically sicker. And so it was directing more resources towards them. And so it was an example of a model embedding a past inequity into the future.
For me, it’s a question of AI for good. You have to be on one side or the other of that argument. If you’re not building for bias and fairness into the models you’re building today, that means you’re building models that reinforce inequities.
The final reason that this comes up to me is that overall it’s sort of a “win, win, win” for society when you address these. Medicare in the US takes care of everybody over 65. And so the healthier we can keep people and the healthier we can keep the population, the better off society is as we go forward because in the end, when people hit 65, Medicare ends up bearing a bunch of those costs. And Medicare means the federal government and the federal government means everybody in the United States is paying taxes.
So ultimately trying to reduce these disparities is very important for not just the health of the people who are being affected, but really also the overall competitiveness and healthiness of the country as a whole.
Maria Palombini
Absolutely. We want to focus on aging healthy. As we all know, the aging population is the fastest-growing segment and it’s going to outpace the younger generation and it’s not just about them living longer, it’s them living longer healthy. So I totally agree. It’s really important.
I wish I would have had you on the first episode of this season, we had a debate on whether AI could help address the issues of healthcare inequity, or some have argued that AI actually makes that gap even wider. The guest that I had agreed with what you were saying, that AI has this opportunity to better address it, but we have seen this debate and it keeps going on. Definitely appreciate your insight on that one, for sure.
Dave DeCaprio
Absolutely. It does have the potential to make things better or worse. It’s all in how we use it.
Maria Palombini
Perfect, almost into our segway about ethics. Ethics means many different things to many different people. Here, we’re talking about in the form of validated and responsible use in the use of AI and/or machine learning for healthcare.
Given your work in various healthcare use cases, what would you like the global healthcare community to know about these types of applications that perhaps they may not be aware or misled when it comes to truly and potentially improving the patient’s health outcomes?
Dave DeCaprio
I think one of the first things for people to realize is that fairness is not a math problem. When you build a model, there’s certain checks you can do to make sure that the model isn’t inherently biased towards one protected group or another, and those are important and straightforward things that you should do. But, don’t take that to the extent of believing that there is a simple report you can run on your model and it comes back with a big green “fair” checkmark or a red “not fair ‘X’” on it.
Anybody who’s trying to simplify these issues that much and tells you there’s something you can run to tell you if your model is fair or not, those people are trying to sell you something. It’s a complicated issue. Fairness is, again, you can’t just look at the algorithm, you have to look at the way that model is being used.
And as an example, you could look at something like racial differences in use of the emergency department. You can go see that different racial groups use the emergency department more or less. It’s unfair to use that information to decide how much you’re going to charge people for health insurance. That’s actually illegal and we say you can’t use somebody’s race to do that. However, if you’re trying to decide which people you should outreach to, to help them, you have an education program about proper use of the healthcare system and when you should go to a primary care provider, and when you should go to the emergency department. You really want to target that towards the right group of people. And so then it may make sense to use that same information for a different purpose. And so fairness, that one model that uses this piece of information, maybe fair when used to determine who you should be educating about proper use of the health system and not fair when you’re using to decide health insurance cost. And so you can’t look at fairness independent of the application of the model. I think that’s probably the first thing that I’d like people to know, and that applies to everything, not just healthcare.
The second point I have is really specific to healthcare and it’s that common fairness metrics often point you in the wrong direction in healthcare. There’s a common fairness metric of quality of outcomes and in some contexts, that’s a very common standard for fairness that’s used in a lot of places and it’s very appropriate.
If we look at giving loans based on gender, we’d ideally say that we would like gender to have no outcome on the outcome of a loan. And we’d ultimately expect in a fair world that the same number of men and women would get approved for loans. Now, there can be a lot of disagreements on if that’s not true, is it really unfair or not? But I think we can all agree that ideally in a perfect world, that would be the right outcome. If you’re building a model to decide who should get breast cancer screening, the answer is not that men and women should get it equally. Breast cancer does actually occur in men. It’s not a zero occurrence thing, but it’s obviously far more prevalent in women.
And so if we’re building a model, a fair use of the model that is trying to figure out proactive outreach around breast cancer, it should be biased towards women. The right answer is that the model should choose many more women than it does men. And so you can’t use these simple metrics that work in maybe a loan decision, in a healthcare context and expect them to get the right answer. And in fact, often they will pull you in the wrong direction.
All of that is to say, you have to think specifically about what you’re doing. There are some good frameworks available to think about these issues. Always consider that health care outcomes can be different than other kinds of outcomes and you need to take that into account when evaluating AI or machine learning.
Maria Palombini
Absolutely. One size cannot fit all and I think that applies to every aspect and every element in what we do in the healthcare system. For sure.
We talked about the great opportunities, the exciting learnings, the innovations, what we’re doing— if you had to think of the most challenging aspect or gap, it could be lack of policy, lack of open data, cybersecurity issues, privacy not addressed right now in these AI applications that continues to maybe cause concern, uncertainty, lack of trust in the tools, what would it be? Why? And in your opinion, what may be the best way to resolve it?
Dave DeCaprio
When we talk to customers and various organizations about AI applications, I think one of the biggest areas that people have is trying to think about the ultimate future they want to get to, and the ultimate vision of what they have and not thinking about the practical steps you can take today.
In a sense. I think all of the problems you brought up, security, privacy, lack of open data, all of these things are major obstacles and they all have big issues that prevent us from the sort of “healthcare data nirvana” that we could imagine. But none of those things means we can’t make progress.
Every one of those, there are practical solutions to get things moving today. I think one of the big things that we see that we try to overcome is to get people to think about what you can do today, with what you have and how to get moving and get practical advantages now. Because the only way we get to that bright future is demonstrating the power of the technology today.
When people start to see that even with all of these barriers confronting us, we can still actually improve decisions we are making today. We have people in Dallas. We have people in Chicago, New York, rural areas throughout the country where we’re making better decisions today using the imperfect data that we already have. And if we can get people to accept that we can start using all of this technology and we can gain trust in it even before everything’s perfect, then I think we can start to move forward and that provides the momentum you need to tackle those bigger challenges.
Maria Palombini
Absolutely. Although I’m going to tell you that I like that term “healthcare nirvana.” I might borrow that from my next webinars series, by the way.
Dave, you shared with us so many great insights. I will tell you that my favorite quote is “fairness is not a math problem.” I might put that on my wall at work. But any final thoughts that you would like to share with our audience? We have many technologists here at the IEEE and beyond our walls who may be already participating in this area of tech in the healthcare domain, or thinking about going into it, any calls to action or things to think about that you would like to impart with them?
Dave DeCaprio
I think I’d say for the technologists considering or interested in healthcare, but who maybe hasn’t been in this space, don’t be afraid of it. It is complex and it is challenging, but it is also very rewarding. It is all things you can learn. It is all problems that can be tackled. So there’s a barrier, but don’t be afraid of getting over that. And then, once you’re there, one thing I’ve already mentioned is humility. Understanding that problems are very large and you can have a huge impact without revolutionizing the system. There are many problems that are broken, find something that’s broken and try to fix it.
And importantly, along with that humility is always remembering, especially for the people who are down in the data that every row in that dataset is a human life. It might be your mom. I always tell people, you can do an analysis and get a result and you might be happy with it. But then what if I told you that row 10 in that analysis, was your mom or row number six was your little brother.
Would you still be happy with it? If you’re in healthcare I think you have the responsibility to ask that question to yourself every time. And every time we present a result or we build a model, we try to think, would I be happy if I knew the output of this model is going to be brought on my mom. And if you’re not, you probably shouldn’t be doing this.
The people in that dataset are somebody’s mom. You need to think about that. So there’s an additional responsibility, I think that comes with health care that you don’t have necessarily when you’re analyzing ad impressions on a click stream. But there’s also along with that responsibility comes the impact you can have and the knowledge you’re actually really affecting potentially somebody’s health and some of the most important decisions they make in their life.
Maria Palombini
Very well said. I think that you just really humanized what you guys are doing with tech. It could be my mom, my best friend, my sister, my brother. And sometimes that gets lost, but I think you just brought it back down to earth.
I want to really thank you for joining me today and having this awesome, great conversation. It’s really been a delight. So thank you so much for joining me.
Dave DeCaprio
Thanks, Maria. It’s been wonderful for me.
Maria Palombini
For all of you out there, if you want to learn more about ClosedLoop, visit ClosedLoop.ai and you can see the awesome work that they’re doing in different areas of applications and their next steps moving forward and what they’re embarking on.
Many of the concepts we had in this conversation with Dave are addressed in various activities throughout our Healthcare Life Science practice here. Our mission of the practice is a lot what Dave referenced today. It’s really looking at how we can support innovation, enable privacy, security, and equitable, sustainable access to quality care for all individuals.
We have projects and initiatives such as WAMIII [Wearables and Medical IoT Interoperability Intelligence, Transforming the Telehealth Paradigm], Decentralized Clinical Trials, Ethical Assurance of Data-driven Technologies for Mental Health Care, and Robotics for the Aging Healthy and Assisted Living. These are all activities people from all over the globe, experts working together, developing standards, identifying situations and challenges. So if you’re interested and you want to learn more about these activities, they’re all open and free to participate. And you can visit ieeesa.io/hls for the healthcare life science practice.
If you enjoyed this podcast and you find the things that you heard today really interesting, and you want to share them with your peers and colleagues, please do so. This is the way we get the information out to the domain, letting them know about the great ideas and all the opportunities and challenges with using these technologies in healthcare. Be sure to use #IEEEHLS or tag us on Twitter @ieeesa or on LinkedIn, IEEE Standards Association when sharing the podcast.
I want to do a special thanks to you, the audience, for listening in today and continue to stay well until next time.
Episode 5
Getting Real about Healthcare Data and the Patient’s Journey
The time has come to unleash the value of unstructured data. Artificial Intelligence (AI) and Machine Learning (ML) afford those opportunities across the healthcare domain, however, AI and ML must be demystified and we need to embrace the value of Natural Language Processing (NLP) in daily operating systems.
Alexandra Ehrlich, Principal Health Innovation Scientist at Oracle, and our host, Maria Palombini, discuss how AI and ML hold great opportunities for healthcare, but we can’t lose sight of the challenge with bias permeating throughout accessible healthcare data.
Speaker
Alexandra Ehrlich
Principal Health Innovation Scientist, Oracle
Alexandra Carolina Ehrlich is a biostatistician with over 15 years of experience in clinical outcomes, clinical trials, and real-world evidence research. She is currently the Lead Principal Health Innovation Scientist with Oracle’s Health Innovation and Scientific Advisory team focusing on novel approaches and solutions for the health and healthcare industry.
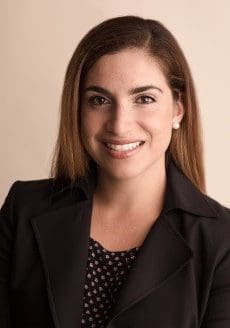
Full Transcript
Maria Palombini
Hello everyone! Welcome to the IEEE SA Re-Think Health Podcast Series. I’m your host Maria Palombini, Director of the IEEE SA Healthcare and Life Sciences Global Practice. This podcast takes industry stakeholders, technologists, researchers, clinicians, regulators, and more from around the globe to task with an important question: how can we rethink the approach to healthcare with the responsible use of new technologies and applications that can afford more security, protection, and sustainable, equitable access to quality care for all individuals? We are currently in Season 3: AI for Good Medicine. If you’d like to check out our previous seasons, please visit ieeesa.io/healthpodcast.
So in Season 3: AI for Good Medicine, we bring a suite of multidisciplinary experts from around the globe to provide insights as to how do we envision artificial intelligence, machine learning, or any other deep learning technology, delivering good medicine for all.
We all want good medicine, but at what price? Essentially, in terms of trust and validation in its use. As healthcare industry stakeholders, we’re not looking for the next frontier of medicine if it’s not pragmatic, responsible and can be equitably valuable to all. And this season, we talk with technologists to clinicians, researchers, ethicists, regulators, and more about how these deep learning technologies can make real and trusted impact on improving outcomes from patients anywhere from drug development to healthcare delivery.
The question is will AI, ML, or deep learning cut through the health data swamp for better health outcomes? So just a short disclaimer before we begin, IEEE does not endorse or financially support any of the products or services mentioned and, or affiliated with our guests experts in this series. It’s my pleasure to welcome Alexandra Ehrlich, Principal Healthcare Innovation Scientist at Oracle.
Welcome, Alexandra!
Alexandra Ehrlich
Thank you for having me, Maria. It’s a pleasure to be here today.
Maria Palombini
Super excited. So for all of you out there, Oracle is a multi-national technology company and one of the top five software technology companies globally. One of its major industries that it operates in is in healthcare.
So today we’re going to talk to Alexandra about going through a realization journey of the challenges with healthcare data, the opportunities to make it better, and where we have many miles to go before we can arrive at that last mile, which we know is the patient. Before we get to the core of the technology and the applications and that kind of thing, we really like to humanize the experience for our listeners. So Alexandra, can you tell us a little bit about you? You have an established background in biostatistics and technology throughout different areas of healthcare and life sciences. What has been the most influential or eye-opening experience in doing this type of work?
Alexandra Ehrlich
It was nice to reflect on that point and to think through some of those eye-opening moments that I’ve had. For me, it was really early on. It was right out of grad school. I was working as a fellow at the CDC, the Centers for Disease Control and Prevention in Atlanta. Coming out of grad school, I had dealt with very clean, very concise datasets, right? So the data that I was interacting with through my learning process was very organized and my first exposure to real life data. We were just analyzing very small components, very small number of attributes. It really hit me then that we have so many answers already locked in the data.
There is a lot of information that is incredibly valuable that for either technological or methodology issues we’re not able to tap into. That really shifted my perspective and my passion from just this insight generation approach into really thinking through the holistic process of data and how to unlock data for a variety of use cases. Even the use cases that weren’t the original use cases that the data was collected for.
So that’s guided my path along the way very early on. Keeping the value of data in mind, not just the primary use but the secondary use of data and really thinking through the systems that enable that.
Maria Palombini
Very important. We’ve had a few guests talk about secondary use of data, and that came up quite a bit actually in this season, particularly. So I see that this seems to be all lining up. Everybody seems to have a very important perspective on that side.
I see that you’re very involved in the Oracle Latinos Alliance. We know we want more diversity in healthcare tech. Can you tell us a little bit about the mission of the Alliance and what are some of the many great benefits that Oracle is doing for the Latino community, both within the organization and potentially outside of it?
Alexandra Ehrlich
Oracle Latinos Alliance have a very succinct goal, which is to empower our members, the Latino community, as well as our allies to really be authentic and to show up authentically in whatever context they’re in, at home, at work with their families and their community.
It’s something that’s really at the core of what we do. And we enable that through different mentorship programs, leadership programs, events, everything from cooking classes that are fun to really deep learning experiences with different guest speakers that we have. But at the core is really maximizing the contribution that every unique individual brings.
And that is not exclusive of their outside life and their history. Many of us are immigrants or parents are immigrants or we’re first generation Americans, first generation college graduates, and really creating a space where we can celebrate what maybe at some points in our lives felt like challenges and see the opportunities in that brings to the table and creating that in a corporate environment is important.
And we have incredible support from Oracle. Oracle has done an amazing job in creating really powerful diversity and inclusion programs across every single group that you can think of. And that collaboration across the groups is also very important. And with that support. It’s not just an employee resource group.
It really translates into initiatives for the different verticals within Oracle, the different diversity councils that really are there to assure that diversity perspective and view is taken into consideration for both business decisions, as well as, decisions for the communities that we serve.
Maria Palombini
Absolutely. I think it’s a great support system for sure. And I think it really speaks to the diversity that’s needed to bring attention, especially in the healthcare life sciences side in health tech. So hopefully you guys continue your success with that. So we’re going to get to the core we know with any new technology and application, there’s always this great deal of buzz on the potential opportunities. And now we’re seeing all this buzz about the use of artificial intelligence, AI, throughout the healthcare system. From your perspective, how pragmatic and realistic are the uses of artificial intelligence or machine learning with healthcare data? Can it benefit the healthcare system today as it stands?
Alexandra Ehrlich
I’ll start with the first part of that question. The comment about it being a buzzword and the important point we’re at right now with AI and machine learning is that we have to survive the buzz, right? Because we’ve seen a lot of trends and fads come and go without really providing, the promised value. We have to de-mystify what AI and machine learning really are. For us at Oracle in our approach and at Oracle Health it’s really been around connecting to the tangible ways that AI and ML is already contributing, is already being heavily used in the healthcare and research and drug discovery areas.
If we think of something like NLP, natural language processing, that is a core functionality of a lot of systems that provides value on a daily basis across different types of providers and people interacting with the healthcare system. So that’s been our approach. It’s that first step is to de-mystify what AI and machine learning are and educating in terms of how it’s currently being used and it’s bringing value in different parts of the industry.
The second part of that, which is can it benefit the health system currently? The answer is absolutely. There’s a lot of areas where we can offload a lot of the workload that is plugging the benefit that humans can bring to the table by leveraging AI and machine learning. This is across the board medical imaging support something like safety for drug candidate compounds, unstructured data, right? Unleashing the value of structured data for different treatment pathways, complex treatment pathways like cancer treatments.
Another place where it’s heavily used now and there’s huge room for it to really expand is the algorithms that are used for symptom detection during emergencies. There’s just so many places where it’s currently providing benefit and focusing on that and showing the tangible ways that we can expand on those use cases has been our approach and it’s really getting traction in the industry and we’re getting such a good reception from providers, caretakers, patients, because it’s something that they can understand. It works in the de-mistifying part of it. They understand how it’s tangible, they understand the benefit and then it simplifies what it means. I think we have ideas of what it is that we can educate and be able to maximize the value.
Maria Palombini
Absolutely. We always think about the algorithm, but there’s so much about the data that’s underneath there that we have to think about as well. You guys are a significant technology partner to the healthcare system. What are some of the greatest challenges you’re hearing from the industry when it comes to data?
Alexandra Ehrlich
Data access. The idea of the right data, right person, right time is something that the healthcare industry has struggled for decades. Once we digitized the healthcare experience, we just had more data we didn’t know what to do with, and we’re still running it. It’s an unsolved problem. We’ve been talking about it for a long time, but every time that we’re speaking to the customers, every time we sit back and reflect on our roadmap as we’re offering solutions, that’s really what we go back to.
It’s kind of the core of all the pain points that we encounter is that data access, that meaningful data access. That actionable data for the right person in the right moment.
Maria Palombini
I think it’s very interesting that you use the term “actionable access” to data because I’ve had two other podcast guests tell me about data not being that valuable if it’s not actionable. So very aligned about some of the conversations that we’re having when it comes to data.
It was interesting. I was actually at a conference the other day and it wasn’t regarding AI, it was actually in another topic, but this seemed to be a major point I keep hearing quite a bit. And it comes up as often from the clinician side and the IT administrators is that the other is trying to make the other’s jobs harder, by always integrating new applications and new technologies and new ideas. And it almost sounds like a communication challenge, however, it just appears the way the data is not designed to flow from one place to another is causing all this extra manual resources and errors and I think you started to touch on this a little bit earlier where machine learning and AI can really save on human resource power, but what can it do to start automating some of this process so that both sides have to work together in implementing these technologies feel or more like each other or feel like they can better work together.
Alexandra Ehrlich
That’s a great question and definitely resonates with our experience as we work with our customers and I’ll address that communication part because I do think it’s crucial. Providers feel like technology is something that’s happening to them and the message that we’ve gotten again and again, is that they don’t feel like they have enough input throughout the process of even assessing the right technology, early on seeing what the options are. So that’s something that is changing, but us as a technology partner, we take that into account and we make sure that we communicate that involvement of the people who are going to be affected by these changes and in the technology that will be implemented. But yes, that is the reality of it and in terms of the way the data is, it really is the nature of the healthcare system.
Different parts of the healthcare system, everything from resource management, revenue cycle management, and then the actual clinical lab systems. They all live in separate places because they serve different purposes, right? So your human capital is going to be consumed in a certain way. The end users are going to be very specific versus your clinical data. That being said, the way that these systems are built, they’re very good at what they do. But when it comes to the more complex questions that would require information across those pillars, that’s where we run into issues. That’s where a lot of the pain points are in the bottleneck to really progressing our analytics. That’s one of the places where AI and machine learning shine. I wouldn’t say it’s a low-hanging fruit because it’s not an easy problem to solve, but it’s that automation. Something like automation of data mapping, discovery of unstructured data to create structure attributes for reporting and analytics, going back to the NLP, being able to mine notes in some of these more complex treatment pathways where the clinical systems are in design to collect that granular information. That’s such a big place of benefit for AI and machine learning that we’re moving towards. We’re trying to stay focused there in terms of the health care industry and us at Oracle Health is how do we really leverage that where it makes sense? That’s really a great place to start for a lot of organizations is to see where they can automate some of that more painful time-consuming processes that humans have been taking for a long time.
Maria Palombini
Yeah, for sure. We touched on this a little bit. We’ve heard data is the new goal, data is an asset, data’s the new oil. We’ve heard so many terms about it. When data sits stagnant, it has less value, right? Not only to the patient, but to the overall advancement of the healthcare system. How can we make data more active and valuable?
Alexandra Ehrlich
We discussed this in the beginning and it’s really around understanding the data and defining what actionable is. Often we define what’s actionable almost in a convenient way with the data that’s available. And then we don’t maximize the value of the technology and the processes. So for us, our approach is really working backwards. What does it mean to have actionable data? What does it mean to really bring value and benefit to the end user? And then we work back from there. The technology’s there. The key is really starting there. What does it mean to be actionable for the people who are impacted by those decisions and then work back from there.
Maria Palombini
Absolutely. I think always keeping the end user, who’s going to be impacted by these outcomes, keep in focus. So I like to do this with all my guests. I call this the think fast question. When I mention, AI for good medicine, what is the first thing that comes to mind and why?
Alexandra Ehrlich
AI in good medicine is giving humans the freedom to do what they’re best at. For me and for us at Oracle it’s really around allowing the humans, the patients, the providers, to be able to connect, to be able to truly be present during that healthcare encounter and AI and machine learning can support that. In many ways across the board. That’s really what I think about when I think about that. If you look at the outcomes, anything that’s related to outcomes across any demographics that provider presence is crucial in positive outcomes and being able to enable that and support that is a huge place for AI and machine learning. A huge opportunity.
Maria Palombini
Awesome. I read one of your recent articles and you mentioned that as organizations reach their digitization goals, they’re facing new challenges. The current healthcare system are generally adequately answering specific questions for end-users, but may be limited in addressing more complex questions. So what types of conflict questions are being restricted and what are some of the opportunities to alleviate those challenges?
Alexandra Ehrlich
In the past few years, and especially in the last couple of years, we are evolving our understanding of what influences health and what influences outcomes. The environment, lifestyle, social-economic status, access, mental health, it’s really beginning to influence how we approach care. And that is creating complexity, from a technology perspective and a data perspective. So as we understand that in order to truly make an impact in someone’s health, we have to take a 360 view of that patient. We reflect on everything that it takes in order to do that. There’s a lot of technological barriers to that. The opportunity to that is the creation of longitudinal views of patients and patients in a healthy state. We usually think of patients only when they’re interacting with a healthcare system, but knowing what this patient’s status is and what their context is when they are healthy, it’s crucial in understanding their prognosis and their options, and their access.
So those are some of the complex questions that are coming our way. And that has to do with everything like wearables, right. And a lot of the IOT and a lot of the engagement platforms, engaging with patients outside of the clinic, everything from the centralized trials and out of the hospital care, all these things are a reality today, escalated and accelerated through the experience we had through COVID. So our systems are catching up to that now.
Maria Palombini
Absolutely. There’s so much more going on today. We often hear about ethics. Ethics in AI in the many different applications. What are the ethical considerations that you see, or you can ascertain that are not getting enough attention when it comes to use of AI machine learning across the healthcare domain?
Alexandra Ehrlich
It’s really understanding the bias in our data. Depending on the bias on your data, it depends who you can generalize to. Who the ending results are applicable for. That’s the starting point and also realizing that this has been a big moment for me in the past year or so. When we look at the training data available to us right now, it’s a snapshot of the present. If we want to create a different future in terms of outcomes, especially around the groups and the populations that are underrepresented, we have to understand that original bias. We’re going to have incredible limitations with the data that exists today in order to create predictive models that will really impact the future in a positive way. Knowing the bias in your data and then understanding that data can only take you so far, because it’s only a reflection of our current state, two components of analyzing the bias and being aware of the bias and the limitations
Maria Palombini
Absolutely. We see this bias discussion keep coming up and it’s something very important that we have to take into consideration as an industry. So for sure.
Alexandra, you’re giving us so many great insights. Any final thoughts you would like to share with our audience, data scientists or artificial intelligence machine learning technologists, working with the data who maybe already operating in the healthcare domain, or is interested in getting into the healthcare domain.
Alexandra Ehrlich
This is something that is a north star for our team and for Oracle Health and something that I communicate as a mentor and as a teacher. You have to understand the problem first. Understanding the problem that you’re trying to solve means that you need to understand what that problem solved looks like for the people who are impacted by that. If that’s your north star and you do your work, your due diligence in understanding that then you work backwards. And a lot of the decisions that you make along the way will be informed by that and should be informed by that. So to me, that’s really what we’re lacking- the deep understanding of the problem and the impact of that problem. And also what a solution truly looks like for those involved.
Maria Palombini
Absolutely. Understand the problem that you’re trying to solve. Some things sometimes you just get so lost in its simplicity.
Alexandra Ehrlich
But in technology is easy to do so because we are creating amazing, powerful technology day in and day out. And for us as technologists and technology partners is something that we have to be very intentional in doing. That’s why I feel very passionate about that.
Maria Palombini
Absolutely. Alexandra, thank you for joining me and sharing all these great insights with our audience and for all of you out there, if you’d like to learn more about Oracle’s work in the health care system, you can visit oracle.com. Many of our concepts in our conversation with Alexandra are addressed in various activities throughout the IEEE SA Healthcare & Life Sciences Practice. The mission of the practice is engaging multidisciplinary stakeholders and have them collaborate, build consensus, and develop potential solutions in an open standardized means to support the innovation that will enable privacy, security and equitable, sustainable access to quality care for all.
Some of our activities include WAMIII, the Wearables and Medical IoT, Interoperability, and Intelligence. That’s a global incubator program and Transforming the Telehealth Paradigm, Decentralized Clinical Trials, and Responsible Innovation for AI for the Life Sciences and a host of other activities.
If you’re interested in learning about these activities and how to get involved, please visit the practice website at, ieeesa.io/hls. If you enjoy this podcast, we ask you to share it with your peers, colleagues on your social media networks. This is the only way we can get these important discussions out into the domain is by you helping us get the word out.
So be sure to use #IEEEHLS or tag us on Twitter @IEEESA or on LinkedIn @IEEE Standards Association when sharing the podcast. Alexandra, thank you for joining us.
Alexandra Ehrlich
Thank you. It’s been a pleasure and an honor, Maria.
Maria Palombini
Thank you so much. And for you, the audience, thank you for joining us and listening in, continue to stay safe and well until next time.
Episode 6
Mind Your Data: The First Rule of Predictive Analytics in Clinical Research
The value of prediction can only be as good as the data it used to make its assumptions. With the growing use of AI, the focus has been more on the accuracy and validation of algorithms, however, we need to get back to the basics— the data. The better the data you put in, the better the insights that will come out.
Aaron Mann, Senior Vice President of Data Science at the Clinical Research Data Sharing Alliance (CRDSA), and our host, Maria Palombini, discuss how open data sharing is paving the way to access more quality, real-world and inclusive data to enable predictivity analytics to be more accurate, resourceful, and utilitarian in the world of clinical research.
Speaker
Aaron Mann
Senior Vice President, Data Science, Clinical Research Data Sharing Alliance (CRDSA)
Aaron Mann is Senior Vice President, Data Science, at the Clinical Research Data Sharing Alliance (CRDSA). Recognizing the data-sharing opportunities and challenges across the landscape, he led the multi-stakeholder effort behind CRDSA’s establishment in 2021. At CRDSA, he is responsible for Work Stream development and delivery and provides subject matter expertise in Data Governance, Secondary Use Standards, Policy Development, Technology Models, and Advocacy.
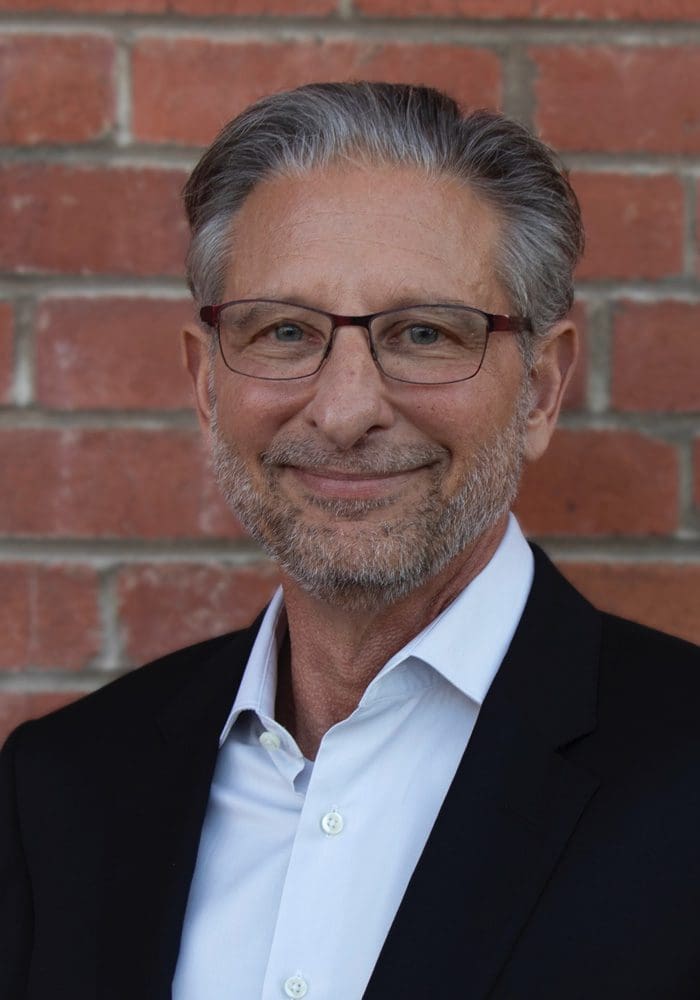
Full Transcript
Maria Palombini
Hello, everyone. Welcome to the IEEE SA Re-Think Health Podcast Series. I’m your host, Maria Palombini, Director of the IEEE SA Healthcare and Life Sciences Global Practice. This podcast takes industry stakeholders, technologists, researchers, clinicians, regulators, and more from around the globe to task. How can we rethink the approach to health care with the responsible use of new technologies and applications in such a way that can afford more security, protection, and sustainable, equitable access to quality care for all individuals.
You can check out our previous seasons of the podcast on ieeesa.io/healthpodcast. Here we are with season three: AI for Good Medicine, which brings a suite of multidisciplinary experts from around the globe to provide insights as to how do we envision artificial intelligence, machine learning, or any other deep learning technology, delivering good medicine for all?
We all want good medicine, but at what price, especially in terms of trust and validation in its use. As healthcare industry stakeholders, we’re not looking for the next frontier of medicine. If it’s not pragmatic, responsible and can be equitably valuable to all. In this season, we go directly to the technologists, the clinicians, the researchers, ethicists, regulators, and others about these deep learning technologies and what real and trusted impact can they have on improving outcomes for patients anywhere from drug development to healthcare delivery.
Will AI, machine learning, or deep learning cut through the health data swamp for better health outcomes? So a short disclaimer, before we begin, IEEE does not endorse or financially support any of the products or services mentioned and/or affiliated with our guest experts in this series.
It is now my pleasure To welcome Aaron Mann, Co-founder and Senior Vice President of Data Science of the CRDSA, Clinical Research data-sharing Alliance. Welcome, Aaron.
Aaron Mann
Hi, Maria. It’s great to be here.
Maria Palombini
So today we’re going to get to the basics- data. Why we need to make sure data going in will give us the benefit expected with predictive analytics and clinical research. Just full disclosure to our audience, the CRDSA is an IEEE ISTO Alliance. ISTO is the Industry Standards and Technology Organization. It is a global 501 C6 not-for-profit offering membership infrastructure and legal umbrella under which member alliances, such as CRDSA, and trade groups can stand themselves up as legal operating entities. For the world out there, you might not know IEEE does have this offering.
Let’s get to humanizing the experience for our listeners. So Aaron, tell us a little bit about you. You have a well blended professional background having been a Program Leader at Genentech, a COVID-19 data-sharing Lead at TransCelerate Biopharma, and prior to that, a CEO of a big data analytics solutions company. As Co-founder of CRDSA, what drives your passion in working with data? What are you hoping to achieve with this Alliance perhaps you felt may have not been realized in prior roles?
Aaron Mann
I think the passion I have for data is what it is and what it represents. Fundamentally it’s people, it’s experiences. Data scientists, sometimes we look at things as a series of data points, but for me, it the fact that those data points represent things that happen in the real world to people and especially in a clinical trial context, when you think a tough study say we’re collecting about 2.6 million data points per phase three clinical trial, but each one of those is a unique part of a person’s experience. I get passionate about what it represents. I think that drives a lot of why I get excited.
From a co-founding CRDSA aspect, really born out of frustration more than anything else. As an ecosystem we get really good in secondary use of data RIAs, data-sharing, talking about the challenges, the problems, what doesn’t work and we’re very eloquent in that. When we started talking, colleagues and I, people representing data-sharing platforms, academic research institutions, and sponsors, we shared this frustration of let’s start talking about solutions. Let’s get eloquent on solutions. Let’s come together and form an organization that can solve problems that we can’t solve in isolation as a single stakeholder or a single platform.
Maria Palombini
Absolutely. When I was talking to my colleagues in the world of blockchain we talked about blockchain for healthcare and blockchain for pharma, the real purists were like, we really have to talk about the data. He goes, we’re not really getting to the core of this conversation. So data seems to permeate all our technologies, no matter where we go.
The CRDSA is an alliance, obviously, and we all see these numerous amounts of consortium alliances that are being formed in many different areas of the healthcare domain. So how is CRDSA different? What is the vision of bringing this alliance together and what are the alliance’s objectives?
Aaron Mann
We spent a lot of time talking to a lot of people before we decided to move forward, to make sure that we first understood the problem and how we might approach it, but also made sure that we were not duplicating anything that’s already out there. It’s important to understand that CRDSA is not a data-sharing platform. So we’re not a data repository, a data lake, but actually data-sharing platforms are members of CRDSA. So our role is to represent the entire ecosystem. We have organizations like CPATH, Project Data Sphere, that are data-sharing platforms, data-sharing organizations, that are founding members, big biopharma companies, technology partners, CROs. We serve as the umbrella organization, looking for solutions that are common solutions to the challenges that we share.
If you really take a step back, the vision is how do we use the type of data that we have to dramatically improve the sharing and reuse of clinical research data and accelerate drug discovery. The easy way to say it, from an objective standpoint, is we want to make it easy to share and easy to use this data. Do we have enough volume going through systems and are we retaining high and updated utility and secondary views?
Maria Palombini
Absolutely. I think that’s a well-blended mix of partners and participants you have in your group. So I think that gives it a really equal voice across the board.
Many times we’ve heard “what you put into it is what you get out of it.” This might hold true for predictive analytics. I spent a good portion of my career, observing and researching the biopharmaceutical medical device industry and I never thought I would hear the words “open data-sharing” in clinical research or anywhere across the pharmaceutical value chain. We all have come to know pharma and clinical- heavy IP sensitive, regulatory complex, and the highest level of competition to get to the next blockbuster.
So can you share with us exactly or what is meant by open data-sharing in the world of clinical research and why this transformational shift over the course of the last few years?
Aaron Mann
We’re in the middle of the transformation. I’m not sure we’ve actually shifted quite yet. We’re definitely on that journey.
I think it is a mind shift that we’ve seen on the part of sponsors and research organizations. Data is not the new oil. That is something that you used to hear a lot more 10 or 15 years ago. It’s not something that gets more valuable over time. The older, yes, the less valuable it is and it doesn’t have any inherent value until you do something with it. I think one thing that’s pushing transformation as senior leaders really getting that it’s about how you use these data and that’s where you’re going to compete. That’s your competitive advantage. Not the actual having of these data.
That leads to a second mind shift, particularly in clinical research, that this is patient donated data. It isn’t something that’s actually owned by sponsors, but sponsors are good stewards of that data. It’s the patients that are coming into the clinical trial setting. They’re donating their time and their data to further the science and reusing that it’s an ethical imperative to honor the commitments that patients have made on the effort that they’ve put in to supporting clinical trials. That shift has happened.
I think the third, and in some ways, maybe most transformational, is advanced analytics- AI/ML. Because it requires big data, right? And as companies start building internal data marts, internal data-sharing capability, they quickly realize that, wow, no matter how big you are, you don’t have enough data or the right data on your own. Even the biggest pharma companies, when you start looking at things like targeted populations in precision medicine will just, you need more. And so that recognition that you can’t go it alone, no matter how big you are is something that I think we’re just on the tip of the iceberg in terms of how deep that permeates organizations. But it’s a shift that we’ve definitely seen accelerating.
Maria Palombini
Absolutely. I think you brought up a really valuable point because for so long we hear data is an asset, but for our accounting friendly people out there, data could be a depreciating asset.
Aaron Mann
I did a slide at a conference once in Las Vegas. I threw it up there like: data is not an asset. Data is an action. If I don’t do something with it, it’s just not worth it.
Maria Palombini
Exactly. It just sits there. Absolutely valuable insight from that point of view. I think this is a simple question, but I’m sure the answer is a lot more complex. Why has it taken so long for clinical researchers or sponsors of clinical trials to realize the potential of the reuse of the data from previous clinical studies? Perhaps the right question could be what exactly was prohibiting them from using it?
Aaron Mann
A little history helps context on this because the sharing of data in a clinical research, secondary reuse sharing is really a pretty new phenomenon. It started in 2013, at any scale, with a number of sponsors coming together with clinical study data requests (CSDR), but that was 2013. That was external data-sharing and data-sharing in that context, a little bit of an unfortunate term, right? Because it always looked to senior leaders, legal, your chief financial officer, like, well, this is us being altruistic and sharing out, but what do we get out of it?
In 2016/2017, you see the rise of that internal data-sharing efforts, and that really brought a sharp lens to what is it that we can do with these data? How should we be approaching it? I think that would have been accelerated, but there was a big monkey wrench that got thrown in about 2018 with the GDPR. It had this uncertainty around what data protection meant. And you saw a little bit of a slowdown where sponsors said, well, you know, I could share these data from previous studies, but am I taking risks when I do it? How do I understand that risk? How do I know what’s acceptable risk?
And so that had thrown a little bit of a curve ball, but I think we now have the tools to really mine these data. I think the rise of AI, machine learning, predictive analytics, advanced analytics tribes has changed. Fundamentally has sponsors now thinking of themselves as data consumers, not just contributors.
But back to your question of what prevents sometimes the open sharing it’s a chicken and egg problem. If their data scientists don’t see enough volume to use an update of utility and external data that they can access and use, then it looks like a one-way street when it really isn’t. It means that a company may not dedicate the resources that are needed to prepare to trials per sharing, make the policy decisions that are going to promote volume and utility.
Maria Palombini
Absolutely. We hear predictive analytics used across multiple industry domains. What kind of impact can it have on clinical research? Are we talking more efficacious clinical studies, more targeted patient recruitment, better meeting enrollment guidelines, all of the above or something different? Maybe you could share with us a case study where you have seen predictive analytics have a significant impact.
Aaron Mann
I think at some level it’s all of the above, but the part that gets me the most excited is the creativity. What don’t we know? When we combine different types of data, clinical research data with RWD, what new therapeutic pathways might be open or what new hypotheses do we generate that we can then go and test? So I think it’s really exciting to think about the things that we don’t know.
In terms of case studies that I’ve seen, there’s a lot being done about earlier safety signal, identification, and classification. It’s an important one. It’s a place where early linkages can be subtle and therefore machine learning, for example, is particularly well-suited to making better predictive models based on early signals that may indicate later significant problems.
The other area that we’ve seen a lot of work being done, particularly around precision medicine is subgroups of population identification and the improvement of targeting inclusion, exclusion criteria, really trying to make the trials fit the use cases and being able to understand better how those responses will play out.
I think when you take a step back, most importantly, an outcome that we see is can we enroll fewer, but the right patients in trials? When we make trials more dynamic, terminate them earlier, where we save time and patient burden, when the predictive analytics are telling us that things may not be going the right way, conversely, moving them through regulatory pathway faster when we see there’s good reason to hit that accelerator pedal.
I think all of these are use cases that have been done and are being done out there. You can’t share the use case specifics problem in terms of being able to share broadly, but lot of work being done in the area and I think a lot of support within organizations for how this can play out and support their drug development process.
Maria Palombini
Those are really great outcomes. Everybody wants more inclusive and diverse populations, but targeted in their trials. So I think that could be a great contributor for sure.
We all know there’s a difference between AI and predictive analytics. However, we know that they share a common challenge. It is this: if incorrect or dirty data goes into it than an invalid or erroneous outcome will come out of it. From your perspective, what’s happening now with the data that is currently being used, that needs to be fixed and how can the work of the CDRSA eliminate or minimize these issues with the data before they’re applied into these algorithms?
Aaron Mann
I think one of it comes back to a volume problem and an access problem. When I talk to AI advanced analytics companies, one of the biggest complaints that I hear is that we’ve built a really good tool, but all of us are training our algorithms on the same sets that are publicly available, same datasets.
So I think there’s a need for more diverse data and data sets that are ready for analysis and have high data utility. We use the word “data utility” at CRDSA intentionally, because it is clinical research data. The good thing is it is collected per protocol with defined outcomes, objective assessments, all of that. So it’s quality data to start with, but it’s going to undergo this transformation for secondary use. That transformation might be to protect patient privacy, it might be to protect IP, but it’s going to go through something before it goes into, for example, an AI tool. That’s the point where you can strip out utility. That’s the volume bottleneck because it takes resources to do that.
So we’re really working on both problems: volume and utility. The way we look at it is it’s going to take movement on policy. Policy at data contributors, its sponsors, and from regulators to be able to bridge that gap of volume and utility and standards around what does good look like? I think all that we’re doing is creating a better data model and data set utility, going into the powerful tools that are being created that power next-generation drug discovery.
Maria Palombini
I’m sure that would be very wanted by a lot of these tool-developers. I like to do this with all my guests and I call it the “think fast” question. When I mention “AI for Good Medicine,” what’s the first thing that comes to mind and why?
Aaron Mann
Creativity. What don’t I know? What hypothesis did I not even think of testing, but because the system, the tool was able to interrogate datasets in a way that generated some new thoughts or insights, I’m able to develop a new way to look at a problem. That’s the exciting part of this and the part that I get most excited about first-line.
Maria Palombini
That’s opening pandora’s box. Opening the unknown. What can we find out? For sure. We always hear a lot about ethics and AI. It’s a big conversation globally. I think it’s in every domain, not just healthcare. When we talk about ethics it’s in the form of validated and responsible use in AI and machine learning for healthcare and I know that the CDRSA has some working groups on patient data governance, data protection, and data ethics. Why is this important in the scope of open data-sharing and what kind of baseline or blueprint are you guys trying to set for the industry to follow?
Aaron Mann
I think there’s an essential tension in data-sharing. On the one hand, all these calls for open science. You hear those calls from WHO, NIH, share open line. But the same organization, like the UN can say, we want to open science and then say, privacy is a fundamental human right, which it is.
And so you have this essential tension between privacy, protecting patients, and open science. That creates this governance continuum in the middle. From a governance data protection standpoint, how you interpret as a sponsor, as a data contributor, how you interpret where you should be on that continuum, determines how much you’re going to share, how much data utility it’s going to have.
We have seen sponsors that have stripped out all adverse events and demographics from a contributed trial. Because they were being very conservative on the patient privacy side without balancing with the data utility side. That’s the exception, not the rule, but it happens out there.
I think it also is around access. How easy is it to get to these data? Novartis is very public about their data42 project internal data mark, and they just published a paper and got to a point where their internal stakeholders can access their secondary use data in almost every case, it’s an automated approval. In contrast, a sponsor I was talking to a couple of weeks ago where their researchers have to put in a formal research request backed by a business case to access any part of their internal clinical trial data. So you have really different sides of that continuum.
For us, what we’re trying to do or give people, sponsors, anchor points to say, this is a way that most people do it, it’s not prescriptive saying you have to do it this way, but this is the balance of acceptable risk, acceptable IP protection that does the best job of fulfilling the ethical duty to protect patient privacy and the ethical duty to share openly and contribute to forwarding the science.
So we’re trying to really create that blueprint or that anchor point that allows sponsors to have a comfort approach that they’ve got is one that is generally accepted best practice.
Maria Palombini
It’s amazing that we still have this conversation about the tension between data-sharing and data privacy. When blockchain in pharma and blockchain in healthcare came out, they’re like, this could be a potential viable mechanism for that and we’re still here talking about it, but I think it’s a very important, valuable point. In another podcast, they were doing a precision oncology study and it was the same thing. Trying to protect the privacy of the patients and what came out during the study was they actually had a suite of patients that they found other conditions in their data that they weren’t even aware about. So they basically had to contact the doctor to tell them, listen, there’s these suite of patients we use for the study that they have this condition and they may not be aware of it. Had the data being completely anonymized, we wouldn’t even be able to go back to their governing physician and say that this problem existed. It’s always that balance. Privacy is great and it’s a human right, but I think you have to sort of balance the costs that potentially might come with it as well and I don’t think anybody has that perfect answer.
Aaron Mann
I think you’re right. The biggest frustration that I see technology companies in this space having, especially here in the US is that thinking GDPR and data protection at that level, it’s a really sobering, eye-opener. You can’t just reuse this clinical trial data as easily as you would think they should be able to. And so I think there needs to be understanding on both sides of what is acceptable data protection and sensitivity to that, as well as open science and bridging that gap. Again, it’s a hard balance. There are a number of companies getting this right, or biopharma sponsors that get this really right. But it’s still a big tension point.
Maria Palombini
Absolutely. We know there’s a lot of vulnerabilities when it comes to patient data. We’re talking about lack of security, every day there’s either a ransomware attack or some sort of hack into a health institution. We have privacy issues, patient data governance structure issues. I know your group is currently working on the development of secondary use standards. So what sorts of issues are you guys trying to resolve through the development of those types of standards?
Aaron Mann
Our focus is on that transformation piece that we talked about. What happens during the transformation? What information is available about it? Right now, it’s frustrating for data contributors because there’s a lack of consistency across platforms, and often they are contributing the same trial across multiple platforms. It’s frustrating for end-users/researchers because they don’t have enough information about what transformations did or will take place to these data.
There’s frustration around the sheer amount of data wrangling that needs to happen if you take trials from three, four, or five different sponsors and trying to pull them into one analytical dataset and find out that you have to do weeks of data management, just to harmonize it enough to start the analysis.
There’s a real opportunity to have standards and accepted practices starting with just transparency. What are you going to get when you get the trial? What is the supporting documentation you’re going to get? What information you’re going to get about what has been redacted down to the variable level. It really doesn’t help when a sponsor says, well, we’ve contributed to trial, we had to redact some adverse events, but because of patient privacy, we can’t tell you what they are. We’ve seen that. And that’s just not helpful because now I’m not sure I don’t know what I to know and it’s really dangerous because I’m not sure whether that redacted adverse event matters to my research question could be central to it. And then if I’m using an irregulatory setting without that traceability and ability to know what happened from the original trial dataset to what a regulator is seeing, step-by-step you don’t have visibility into that makes it very difficult to use it in a regulatory setting.
So our mission in secondary use standards is to start bridging that gap first by transparency on the transformations, and then moving through issues and challenges like data harmonization ultimately all the way through increasing the utility by having standards for how data should be transformed.
Maria Palombini
Fascinating. Wow, Aaron. You’ve given us so many great insights. I’m sure the shockwave was, data’s not an asset. Let’s call it an active ingredient for clinical research insights, but just for our audience, maybe you want to share a final thought, could be a call to action for data scientists or data ethicists, AI technologists working with the data who may be in this domain or interested in pursuing this area to support clinical research innovations.
What would be your call to them or parting word of advice?
Aaron Mann
I think if you’re with a biopharma company, if you’re on the data management study side, be good stewards of the data that you have. Share it readily and well, and remember that you’re competing on the analysis, not the data. If you’re on the research side, the data science side, you’re a biostatistician, understand that it’s there, it’s a competitive mandatory. Seek it out. Because from an organizational standpoint, there’s no better reason that your organization, your company will share and participate than if you’re biostatisticians your data, scientists are active users of these data. And I think on the other side, if you’re an AI advanced analytics partner technology company, I think that to know is firstly, the data’s out there, your specific client, a large organization may not know it’s there, but it is. It is a real opportunity to push the competitive advantage of using particularly data external to an organization effectively.
So I think it’s a real opportunity for the technology companies to be an agent of change and drive awareness and a mindset shift within particularly large biopharma organizations.
Maria Palombini
That’s really important. Special thanks to you for joining me today and sharing these great insights.
Aaron Mann
Fantastic. Thank you so much. It’s a great opportunity. Thank you again.
Maria Palombini
Absolutely. I could have talked to you on two other topics and take this podcast for a few more hours, but if you want to learn more about the CRDSA or how to become a member of the alliance, visit crdsalliance.org.
Many of our concepts in our conversation with Aaron are addressed in various activities throughout the Healthcare and Life Science Practice.
The mission of the practice is really engaging multi-disciplinary stakeholders and have them collaborate, build consensus, and develop potential solutions in an open standardized means to support innovation, ultimately helping to enable privacy, security, and equitable, sustainable access to quality care for all.
Activities we are in: wearables and medical IoT, transforming telehealth, decentralized clinical trials, mental therapeutics for healthcare, robotics for the aging. There are many different areas and they’re all touching an element of AI, machine learning, and the work they’re doing. If you want to get involved, visit ieeesa.io/hls.
If you enjoy this podcast, we ask that you share it with your peers, your colleagues, or on your social media networks. This is the only way we can get these important discussions out into the domain by you helping us to get the word out. You can use #ieeehls or you could tag us on Twitter @ieeesa or on LinkedIn @IEEE Standards Association when sharing this podcast.
I want to thank you, the audience for listening in. Continue to stay well until next time.
Episode 7
Can the Health System Benefit from AI as it Stands Today?
With the focus on accuracy, ethics, and bias in AI algorithms, we cannot lose sight of the need for more validated data. With hard-hitting insights and references, is the right question being asked: is AI good for medicine or is medicine right for AI?
Dr. Dimitrios Kalogeropoulos, Senior Independent Consultant for organizations like the World Health Organization (WHO) and the United Nations International Children’s Emergency Fund (UNICEF), looks to the data for answers with our host, Maria Palombini.
Speaker
Dimitrios Kalogeropoulos, PhD
Senior Independent Consultant, WHO & UNICEF
Dr. Dimitrios Kalogeropoulos is a global health innovation, health systems governance, and data ecosystems consultant recognized by peers worldwide as an industry leader and key policy expert for equitable, value-based health care, enabling and strengthening collaboration, engagement and learning health ecosystems, clinical research, and clinical economics. Being an expert with the World Bank, European Commission, UNICEF and the WHO, he has significant global experience advising on decision pipelines, data ethics, health tech, and tech-driven policy, including governments, think-tanks, multilateral and bilateral international development partners, and philanthropic organizations.
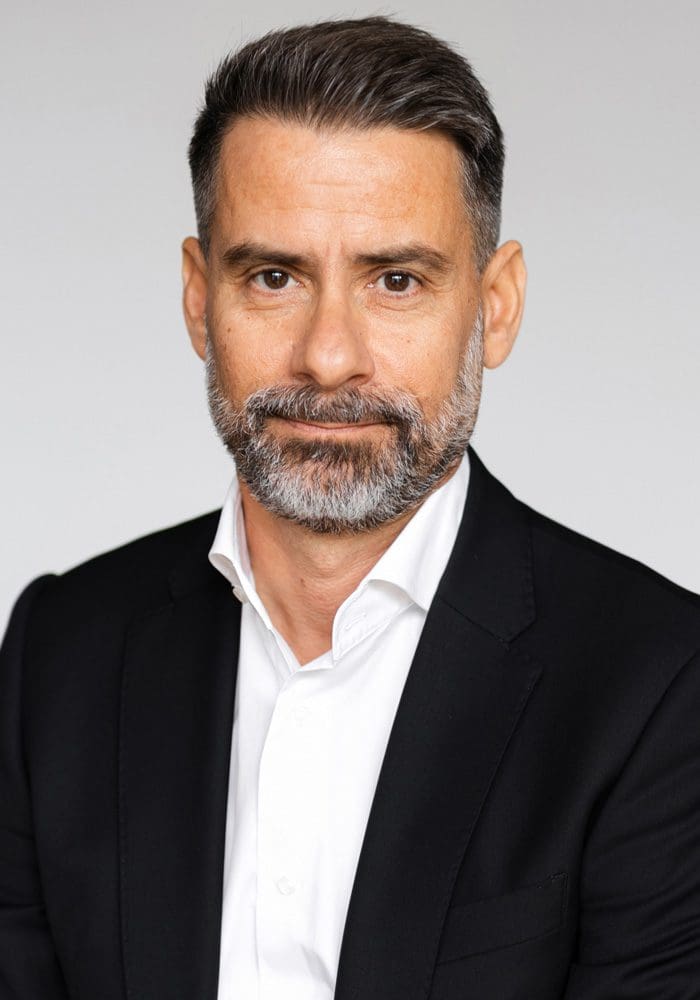
Full Transcript
Maria Palombini
Hello everyone. Welcome to the IEEE SA Re-think Health Podcast Series. I’m your host, Maria Palombini, Director of the IEEE SA Healthcare and Life Sciences Global Practice. This podcast takes industry stakeholders, technologists, researchers, clinicians, regulators, and more from around the globe to task. We ask: how can we rethink the approach to healthcare with the responsible use of new technologies and applications that can afford more security protection and sustainable, equitable access to quality care for all individuals?
We are currently in season three. You can check out our previous seasons on ieeesa.io/healthpodcast. So with season three, our theme is “AI for Good Medicine,” which brings a suite of multidisciplinary experts from around the globe to provide insights as to how do we envision artificial intelligence, or machine learning, or any other deep learning technology to deliver good medicine for all.
We all want good medicine, but at what price, essentially, in terms of trust and validation in its use. As healthcare industry stakeholders, we’re not looking for the next frontier of medicine if it’s not pragmatic, responsible and can be equitably valuable to all.
So just a short disclaimer, before we begin, IEEE does not endorse or financially support any of the products or services mentioned and/or affiliated with our guest experts in this series.
It is now my pleasure to welcome Dr. Dimitri Kalogeropoulos , who is Senior Independent Consultant in global health innovation, digital development, and governance and policy for organizations, including the World Bank, the European Commission, the World Health Organization, UNICEF, and more. Dimitri, welcome to our conversation!
Dimitrios Kalogeropoulos
Hello, thank you, Maria for the welcome! That’s correct. 20 years in international development and global health innovation and 30 altogether in the field of measurement and information in medicine and AI.
Maria Palombini
In this season, we go directly to our technologists, clinicians, researchers, ethicists regulators, and more about how these deep learning technologies can make real and trusted impact on improving outcomes for patients anywhere from drug development to healthcare.
The question is will AI machine learning or deep learning cut through the health data swamp for better health outcomes?
Dimitrios Kalogeropoulos
Let me start by putting things into perspective, before I answer your first question. Prior to COVID-19, digital health was not, let’s say, a public utility. Instead, it felt as if you were in the luxury watch business, then overnight, everything changed. The world now perceives health tech as a necessity, the path to universal health coverage and has set out to discover how to get there. Am I going to do the things embarking on the famous ethics and governance journey? Which is luckily, beginning to read some critical junctures. We get that with that, the challenge is different now. Going digital is all about addressing both local and global challenges. The latter is now the case more than ever before. For instance, our audience might know the EU has developed a financing instrument for this purpose, called the Global Challenges Program of the Neighborhood Development and International Cooperation Instruments for Global Europe and has established mechanisms to strengthen collaboration with other global challenges, programs, such as the Vaccine Alliance, better known as Gavi.
Connectivity has obviously gone global, so how are we faring then? Before the pandemic, a global challenge was to report mortality and morbidity statistics using WHO’s, by now 11th revision, of ICD. And to compare these figures on the basis of national statistical compendia. This was also, world’s kind of common definition of interoperability and interoperable data, and that’s not long ago.
What happened then is that while being fairly inexperienced, all of the sudden we literally went online and sit there. In the meantime that we had been using technology for more than three decades to reinforce how transactional models of reality, the society at large and the economy. And then all of a sudden we woke up to a new virtual reality of real-time digital interaction and task boards.
And so interoperability stopped being theory and became a headache. As a reflex, almost, we pulled out anything we could get hold of to show the world we’re ready to respond and be responsible about it all, including our decisions concerning COVID-19. And we could no longer make decisions the way we used to. Now, evidence is more important than ever before. Since the 2018 World Health Assembly Resolution on digital health, which called for a demonstration of a tighter integration of health systems strengthening with digital health, including our global crisis responses. Quite simply, this resolution means that embracing digital health becomes more normative and thus more scientific.
And this is where my background kicks in— science and ethics in medical decisions and data in order to build responsible and accountable health systems that deliver and promote equitable, affordable, and a universal access to health for all. This is underwritten by a desire to change healthcare and medical research in order to make access more democratic.
Now, to make it all clear, none of this has anything to do with how we finance, but how we use financing for the equal benefit of patients and society at large. Now as for achievements convincing prior to the pandemic, one of the largest multilateral international development organizations to adopt this approach, not on one, but on two occasions in central east Asia, and to move away from the siloed tech understanding of it all, that, in my opinion, is my greatest achievement.
It sounds underplayed, but what has to be in the game to the standard crafting policy is not part of the deployment of international development funds, not before the pandemic anyway.
Maria Palombini
Wow, Dimitri! That’s such a powerful opening statement. Right away you can tap absolutely your passion in this area and all the great work you’ve done.
We often hear, Dimitri, there’s this intrinsic value in the healthcare system. And although that’s true, it has to be instrumental as well. So we have these technologies such as AI, machine learning to extract that insight, but yet we still seem unable to truly rely on it.
So the question we are going to face and get through with you today is how can we make the tides turn the right way? You and I know AI, there’s this great buzz. We see it everywhere. So many different potential beneficial opportunities throughout the healthcare system. But from your perspective, how pragmatic and realistic are the uses of AI in healthcare? Can it and does it benefit the healthcare system today in its current state?
Dimitrios Kalogeropoulos
With regard to what I’m seeing, a moment ago, I brought up the significance of the World Health Assembly Resolution on digital health toward enabling a future where technology serves good medicine and good health for all.
But the question is four years down the line, and after a deadly pandemic, have we learned any lessons? Is health tech now understood as the means to directly influence better care, or is it still seen as a tool for analysis and statistical reflection? What progress have we made toward enabling trusted data sharing for digital diplomacy for value-based care in economics and pragmatic clinical trials?
All these are major targets, but unfortunately, to date, I’m afraid, I believe we have made very little progress towards these goals. Not so much in terms of results, this will follow, but in terms of changing our mentality, when we think health. Instead of enabling a circular economy in health innovation, we are still tapping into whatever pool of data we can get hold of.
Only now, we use AI to feed other AIS, hopefully with reliable data and then evaluating if our data is in data reliable. Confusing, no? It is. One key question is why not make data reliable by design? Make the data trustworthy. Another key question is why don’t we make trusted data available on tap to make the data accessible for any use, without doctoring, cleaning it and curating it as we do currently to develop artificial intelligence. On top of this one also needs to consider the higher demand for data, means a hard data production factor with industry innovation. Instead of a great restart, we ended up with a great pile up of data.
Now in my opinion, we’re still turning away from the problem of data or the elephant in the room. And this is because it is a complex one to solve. It is almost political and we don’t really want to invest in its solution. To give you an idea, I was chatting with a friend recently who is very active in European health innovation. So this friend says to me, to attract funding, you need to demonstrate a clear purpose in terms of the problem being solved. Right? Well, it makes sense. For example, come up with a treatment for cancer. If only it were that simple because we’re seeing progress and hope in this domain and in MS, but through the use of new vaccination technology. And that important association is not that clear after all. Simply stated investors think of market innovation rather than system change platforms or sustaining innovation. And right there lies the core of the problem in this capital misconception of the birth of disconnected, but seemingly focused innovation will magically get us to some ideal future that we know little about.
To give you a clue, at 2021 Open Data and Action OACD Study conducted on early initiatives during the COVID-19 pandemic, found there has been a missed opportunity to use data to address the multidimensional implications of the pandemic with sophisticated enough products and services. Well, that went by very fast. Other studies have made this abundantly clear too, indicating that the lack of access to proper data led to a lack of governance. Data arriving slowly in a rapidly changing situation with empty data fields and with passing-the-bucket processes being the norm. And this report comes from the US.
So let’s face it. Health data is still an abyss, which is why I think it is too early to worry about the consequences of navigational autonomy when we talk about ethics. What we need is autonomous data.
Now the second part about how pragmatic and realistic the uses of AI in healthcare are well, in a nutshell, there’s a huge potential for significant benefits.
The largest benefit will come from enabling trusted data sharing because AI supported clinical processes must be trusted, cost beneficial, in terms of the alternatives or competitors and ultimately clinically effective and efficient. But since the latter also depends on the patient outcome oriented utility of each innovation, rather than an absolute performance part, we have to be clear about what we are expecting.
Consider for example, COVID 19 vaccines. They performed relatively poorly in terms of stopping infection and transmission, but they’re very good in terms of stopping disease progression, and mortality. And this means that we are allowed room for the latter herd immunity to be developed in due time, but not with the vaccine alone.
So this vaccine works like a stent, very similar with current AI applications. Let me give you one example. I recently read an article in the European Heart Journal on an AI tool for the detection of aortic stenosis from test radiographs.
Now, the study showed that AI could detect stenosis in 83% of the cases. We might consider this 83%, not enough. Well, it all depends. What is the average rate of detection, for example, without artificial intelligence, what is the purpose of the tool and what is the evaluation endpoint?
The artificial intelligence would certainly not be patient-facing in this case, a doctor would use it. So then this 83% perhaps is good enough in actual fact. So comparative analysis can be very illuminating when we judge the performance of artificial intelligence.
There’s another example of an AI, recent evaluation for cancer screening that showed the relative reduction of colorectal cancer of 4.8% and the mortality reduction of 3.6%.
And that sounds very low if the data are accurate, but we have to look at the significance from a wider angle, the significance of these results, because it was estimated that the decrease in costs per screened, individual led to an estimated savings of the order of US 290 million at the use population level.
So now that starts making a lot more sense. The real issue here is that the kind of effort required to develop these tools. The effort required to repurpose the tools and update them when bias is detected and the effort required to integrate the tools into clinical practice. Well, it’s quite high. The efforts to carry out these tasks is rendering the deployment prohibitive in terms of the overall cost-effectiveness of the endeavor.
So we need to invest in this problem too.
Maria Palombini
Okay. Dimitri There’s a major focal point of AI machine learning about how accurate are the results from the algorithm. The impetus has placed on the algorithm, but what about the data? What are we not addressing when it comes to the data that is being utilized to train these algorithms?
Dimitrios Kalogeropoulos
It is estimated that the machine learning project must invest 80 to 85% in curating data sets, make it reliable. And then we have to deal with explainability, interpretability, and a host of other issues attached to their available data sources. Our data sources are simply not up to any acceptable reliability standard. Not when decisions are automated with tools integrated in the clinical environment and therefore have to rely on machines to process things like ground truths and diagnostic gold standards instead of actually just feeding them.
But how can we expect any standard of data to reach our AI and other innovations, our research, our decisions, when we know nothing about the origins in quality of this data? We know we cannot trust data and instead of making it trustworthy before it goes up there, in the vast subspace of global health data, we devise instruments, secure it again, we’re seeing the treatment before prevention pattern.
After all, old habits die hard. Innovators have even produced AI with Scouts, the ecosystem for proper data resorting to the use of synthetic rather than real world data. But what happened to the original aim behind big real world data or big data? Well, take a deep breath and imagine deep fakes in health. Scary. Right?
So with all that, we’re essentially rebranding the data issue as an outlier to skew progress completely in the wrong direction and in order to avoid the least attractive of all innovations, that of sharing data. Data about the pandemic and how we may strengthen our health system to deal with the next one should not require a global operation of the scale and scope conducted by the WHO to get hold of, here I’m referring to the excess mortality study, which required vast resources to produce valuable insights. These kinds of insights should be available on those passports that became so popular because of the pandemic, a new kind, which tells you what to look out for when you have X or Y comorbidities and which medicines to avoid as a result.
The bottom line is, it is time we started using data to its true capacity to save lives and improve access to care. And with the new wave of AI, I see both an opportunity to change that as well as a huge risk that we waste the opportunity in all it’s grief, because we don’t understand the extent or exact nature of the stale data predicament.
Maria Palombini
Interesting. I know you mentioned this before: data is an abyss and there’s been so much focus on data and that data is an asset. However, like anything else when data sits stagnant, it has less value not only to helping the patient, but the overall advancement of healthcare. How can we make data more “active” and valuable? Is it something like more open data sharing? Could it be better integration to clinical care, better integration with technologies? What is your perspective on how we can make data more active and valuable?
Dimitrios Kalogeropoulos
All of the above. To make data more active and valuable, we need to adopt the recommendation made in the World Bank’s 2021 Flagship Report: Data for Better Lives. The model it proposes of value, equity, and trust as the social contract with data. With that, we need to build up policies and roadmaps for these still development in health in order to provide for directionality and still the implementation of this social contract. Last but not least, technology policies have to match our normative governance frameworks and institutions must adopt to embrace new horizon scanning and portfolio-based system chains approaches, which are underwritten bioscans, ethnographic research and much more. Regulatory tools such as GDPR are important, but we need to keep in mind once we start encouraging the flow of data, we need to have the mechanisms in place to safeguard trust in the data too. And this, despite appearances, is far from being on the table as a key issue.
We also need to keep in mind tools, such as Software as a Medical Device, in the U.S., or they use MDR and GDPR albeit very important, are extremely inefficient for changing the tide. We need to call it out. We have been wrong about what interoperability means and entails. A little food for thought, how are we going to implement the all important quaternary prevention operations that public health needs? And we are lacking and relevant AMR, Anti-microbial Resistance, policies without interoperability and this to name, but one major pain area.
Maria Palombini
Wow, that’s a very insightful point; a good question for our audience to start thinking about. You know, I like to do this with my guests. I call it the “think fast” question. So here it is. When I mention “AI for Good Medicine,” what’s the first thing that comes to mind and why?
Dimitrios Kalogeropoulos
Good medicine for AI, because digital health is a mirror.
Maria Palombini
Interesting. There’s something insightful to think about. We talk about ethics in AI for various important reasons and we talk about it in the form of validated and responsible use for healthcare. From your perspective, what are the ethical considerations that are not getting enough attention when it comes to the use of these types of technologies in the healthcare system?
Dimitrios Kalogeropoulos
This article I read quite a while ago about how understanding racial heritage can save lives. I was very alarmed with that article, and I think it’s very relevant because this is about the ethics of data, algorithms, pathophysiology models, and relevant decision-informing devices like AI. Also about the collapse of ethics when digital development in health and vast data is not inclusive leading to racial, gender-based, or other forms of bias.
Now this thought article I mentioned presents a case about how early stage chronic kidney disease is similar across racial and ethnic groups. Black people are almost four times more likely than white people to develop end-stage kidney disease and how racially tilted estimated GFR markers have been causing thousands of black people with kidney problems to wait longer to get on the transplant list. Only to now discover that the race-based calculations used in the U.S. after 1999, misled patients and their doctors to believe their kidneys were working better than they really were. Also affecting decisions about medications, diets, lifestyle that could have worsened kidney damage or created other medical risks. Now consider, this formula and something much larger than this would go in a decision-informing system. The consequences could be dire.
Every year, the Stanford Institute for Human-Centered AI compiles an AI index that sums up the state of play in AI, this year in a whopping 190 pages. So I recently read an IEEE Spectrum summary of 12 charts, making some basic inferences of my own to capture the state of play from the ecosystem ethics perspective and here they are.
Number one, investment in AI is off the hook with a number of financing rounds climbing. So single projects attract more financing than new projects do and this is not necessarily a good thing.
Number two, there is still a disconcerting gap between corporate recognition of AI risks and attempts to mitigate those risks.
Number three, AI vision has reached a plateau, which means we need to look elsewhere for progress, perhaps something wrong with our data.
Number four, reasoning is still a frontier of AI.
Number five, ethics everywhere.
Number six, the legislature is paying attention.
Number seven, the carbon footprint of the current AI pipelines is finally being noticed, which begs the question, hasn’t anyone heard of digital recycling?
Number eight, the data ethics problem is clearly still the elephant in the room.
And finally number nine, AI needs women as men are clearly bad at building AI.
We don’t have enough creativity, obviously in artificial intelligence. There’s a point later on, I want to come back to, with regard to bias in developing systems and actually running them afterward. These are the ethical considerations that we need to pay more attention to.
Maria Palombini
Wow. That’s a very strong, I would call it a top 10 list. Something to definitely think about and really a lot of areas like the digital recycling not being discussed or addressed at least as pervasive and important as it should be right now. So very important insight. My next question is about the vulnerabilities when it comes to patient data. In your opinion, what are some of these threats? I think you’ve just outlined some really hard-hitting ones. Where do you think potentially global technical or data standards may be of important consideration to maybe help resolve some of these issues?
Dimitrios Kalogeropoulos
Vulnerabilities, they haven’t changed. The threats haven’t changed and when I say they haven’t changed I’m referring to the past 25 years. Let me quote part of one of my PhD publications, which was written 25 years ago. I quote “the rapidly disseminated practices of evidence-based medicine and outcomes-based medicine or disease management concepts, which were born and developed within the realm of measurement and information in medicine and associated technologies have led to the proliferation of quite a number of approaches to clinical decision-making support. Some of these include the use of advanced IT while some other have negligently avoided the use of the underlying enabling tools. Evidence-based clinical guidelines and care pathways are, but a taste.” Doesn’t that sound current? In many ways, we’re still doing exactly the same thing that we were doing 25 years ago. A lot of potential, very little application in real life. This is a major threat.
So as proud as I am for having conducted research sets two decades ago, I’m astonished that two decades later, we still have to deal with the same impediments to achieve progress in the transformation of our health systems to patient-centered systems through digital enablement and support.
I’m optimistic, nonetheless, that we are not going to wait another two decades as great achievements are being reported in terms of digital in the service of new grassroots, social governance models and change in other sectors. Together with blockchain and other, by now not so much frontier technologies, new superhighway change path forms are being delivered to morph and influence the future we all envision for our health ecosystems. Sure, there’s hype in there too. So what?
Now, as far as standards go, there are plenty underwriting data interoperability, such as as ICD-11, 80-C, LOINC, SNOWMED Clinical Terms, with their application utility in the context of COVID-19 cancer outcomes, classification, and other new knowledge and data classification domains, constantly expanding.
Then there is FHIR with 807 covering a lot of the ground from basic messaging interoperability to discrete data set modeling within messages. Fast Health Interoperability Resources is what FHIR refers to, but we need much more to reach a full set. We need to cover Structural and Organizational Interoperability or SOI and there we lack significantly. By SOI, I’m referring to phenotyping genotypes, enabling the clinical applications of precision medicine, building ethical AI, but doing away with the need to provide handwritten annotations in order to frame the genotypes or to engineer ground truths. I believe we have all heard the case about AI detecting as a diagnostic pattern that octopus ink signature in annotated images and this is something that we have to stop from happening if we are to trust these devices.
Let me add one more experience. A few years ago, I was consulting with a group from a technology savvy country in an international development project. This group reacted strongly to my proposal to use ISO 3940 Standard in the core set, which is by the way, they only, as well as a very mature, SOI standard and Structure and Organization Interoperability standards for clinical data modeling in support of continuity of care. To my great surprise, I recently heard that the particular group is studying the application of the standard in their home country. So the message here is clear. Keep an open mind, think outside the box for cohesive collaboration themes and make digital development far more efficient than it currently is. Because in terms of implementation capacity, we are currently struggling significantly behind the regulatory front runner or rabbit.
Maria Palombini
Wow. That’s great insight. I think you’ve given us so much today. I think with every single response, there’s been some call to get a reference site, to think about different ways of a situation. For our audience, this has been very helpful. My final question to you is, are there any final thoughts you would like to share with our audience? We have a very broad audience. It could be technologists, clinicians, regulators, researchers. Is there a call to action potentially for a data scientist out there or an AI technologist who’s working with the data, may already be in this domain or is interested in getting into the healthcare domain? What is your final imparting thoughts to them?
Dimitrios Kalogeropoulos
Yes, Maria, there are three things I would like to mention. The first one is thinking at the crossroads of leadership and innovation, data governance, that equity and inclusiveness in design teams and design thinking will breed equitable and inclusive designs. This is what I mentioned earlier when I said that women and men are not equally participant in the AI development process. This is probably the most important message technology can deliver for learning health systems that truly empower the patients. To think outside the box, to think without the box in healthcare, we need to empower and engage patients and the community as the teams that will design tomorrow’s equitable and inclusive health systems. And for this gaining the trust to share data is of paramount importance. Trust that will be used to create health systems that learn how to be equitable and inclusive.
With regard to decision scientists or data scientists, I understand that in 1996, the International Federation of Classification Societies became the first conference, specifically featured data sciences and topic, also the year that I completed my PhD research. Now with data science being recognized as a field of science and application, we need to expand our perimeter to safeguards trusted information engineering. Data science needs to push its boundaries and to close the loop from data to knowledge and back to recycled data, to support longitudinal data and to protect the temporal and semantic value of clinical data for providers and for society at large.
Last but not least, the ultimate secret is if keep eyes on the integrated care crosshairs. Focus on concepts like bundled services and value based outcome oriented clinical decisions to reveal the path to longitudinal data, provider interoperability, and trust the data sharing. Remember that digital health is a mirror. In the process, think big, but always start small.
Allow me to also add that further information can be found in articles that are published on LinkedIn Pulse and with that, thank you for the invitation Maria and for being such an excellent host. I hope we get to chat again in one of your upcoming seasons of Re-think Health. Thank you.
Maria Palombini
Absolutely. Dimitri, you’ve given us so many great insights. Our next season is going into telehealth, but a lot of the points you brought up today definitely refer to that whole new paradigm of patient-centered healthcare. And so, to all of you out there, many of the concepts in our conversation with Dimitri today are addressed in various activities throughout the IEEE SA Healthcare and Life Sciences practice.
The mission of the practice is engaging multidisciplinary stakeholders and have them collaborate, build consensus, and develop potential solutions in an open standardized means to support innovation that will enable privacy, security and equitable, sustainable access to quality care for all.
Some of our activities include WAMIII (Wearables and Medical IoT Interoperability Intelligence), Transforming the Telehealth Paradigm, Responsible Innovation of AI for the Life Sciences, and a host of other areas all across the healthcare life science domain. If you’re interested in getting involved and learning more about the programs I mentioned and the others that are in our activity list, please visit ieeesa.io/hls.
If you enjoyed this podcast, we ask you to share it with your peers, colleagues on your social media networks. This is the only way we can get these important discussions out into the domain, by you helping us to get the word out. Be sure to use #ieeehls or tag us on Twitter @ieeesa or on LinkedIn @IEEE Standards Association when sharing this podcast.
So to you, the audience, a special thank you for listening in. Continue to stay safe and well until next time.
Episode 8
Advanced AI & Sensors: Reaching the Hardest to Reach Patients at Home
Healthcare is coming home. Sumit Nagpal, CEO & Founder of Cherish Health, explains how using advanced AI and sensors can efficiently and effectively support the wellness needs of the rapidly growing elder generation at home with dignity and integrity.
Speaker
Sumit Nagpal
CEO & Founder, Cherish Health
Sumit is the CEO and founder of Cherish Health. Cherish Health develops advanced sensors and artificial intelligence, combined with medical evidence and human touch, and uses these to provide solutions for people aging or living with health challenges – our grandparents, parents, children, many of us – that help improve their lives and support their self-care. Sumit also serves on the HIMSS Enterprise and Health eVillages boards and is sought after for his expertise and unstoppable energy as an entrepreneur, change agent, strategist, and technology architect.
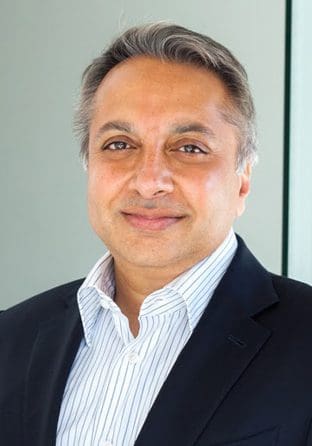
Full Transcript
Maria Palombini
Hello, everyone and welcome to season three of the IEEE SA Re-think Health Podcast Series. I’m your host, Maria Palombini and I am the Director of the Healthcare and Life Sciences Global Practice. So our practice is a platform for multidisciplinary stakeholders from around the globe to collaborate, explore, and develop solutions that will drive responsible adoption of new technologies and applications leading to more security protection and sustainable, equitable access to quality of care for all individuals.
Yes, a very ambitious goal, but a very necessary goal. The Re-think Health Podcast Series brings awareness to all of these concepts and a balanced understanding in the use of all new technologies and tools and applications where we may need more policy or standards to drive this responsible, trusted, and validated adoption to enable better health for all. All of our seasons and our podcasts are available on Podbean, iTunes, and other podcast providers.
Season three is titled AI for Good Medicine. And it’s not just about AI. We’re looking at machine learning, artificial intelligence, deep learning technologies, and really looking at how these multidisciplinary experts from around the globe can provide insight as to how do we envision this AI/ML delivering good medicine for all?
The reality is we all want good medicine, but at what price and price really here means in terms of trust and validation in its use. As healthcare industry stakeholders, we’re not just looking for the next frontier of medicine if it’s not pragmatic, responsible and can be equitably valuable to all.
And so we go deep with the technologists in this season, we talk to the clinicians and the researchers and the ethicists and the regulators, and really trying to understand what can be real and trusted impact on using these technologies for improving outcomes for patients everywhere. The reality is can this really help us cut through this health data swamp and deliver better outcomes?
And with that, I like to welcome Sumit Nagpal to our discussion for the true potential of AI in healthcare, helping marginalized populations or helping those, even in the form of the elderly. All these populations that, yeah, they may have access, but exactly how do we reach them in the right way?
Sumit is a serial entrepreneur with a focus on digital health innovation at scale. He has co-founded and grown five companies over the past two decades and has tackled progressively bolder challenges facing our healthcare economies. All his work features common themes: big, bold ideas to help us imagine a better world, incredibly complex processes into simple, approachable and engaging user experiences, implementation models that blend big innovations into the fabric of our daily life. He has honed since the time he has worked with Steve Jobs and NEXT in the early 1990s.
Sumit, welcome to the Re-think Health Podcast Series.
Sumit Nagpal
Thank you so much for having me.
Maria Palombini
I think you have an exciting background, just reading this very short descriptive paragraph. You’re a serial digital health entrepreneur. It’s an opportunity to work with some great tech gurus and renowned companies. Can you share with us what has been the greatest reward and maybe some of the greatest challenges that you’ve confronted?
Sumit Nagpal
Awesome way to start, thank you! Let me start with the greatest reward. It’s the chance to work with smart, motivated, creative, innovative people every day on shared missions. To make a real difference in people’s lives. I’ve been very lucky to have coaches, mentors, colleagues, clients- my heroes who keep me on my toes, challenged me to be and do better, and to be super clear about why we do what we do.
I recently told an emerging partner of mine: the past eight weeks of working with him have felt like yet another MBA. I’m super lucky to wake up every morning, knowing that no matter what, I will get to work with incredible people, grow personally, and help move the world forward. What more could anyone ask for?
Regarding challenges I’ve learned over the past several decades, perhaps too slowly that sometimes the world isn’t ready for even the best ideas. I started a company focused on digitizing medical records before we knew that those would be called electronic medical records.
I started another company focused on joining up people’s healthcare data from wherever they receive care. It’s taken 20 years for that to be legislated into existence. So being able to imagine the future and what’s possible, sometimes that’s called being ahead of your time. Sometimes that’s also called being dense.
That’s both a great challenge and a great learning experience for me.
Maria Palombini
Absolutely. I may have run into this a few times, myself. You know, it’s always exciting to be above the curve and you wanna get to the next emerging thing, but sometimes the world is not ready for it yet. And so there’s always a right place and the right time for everything.
Now you’re in this world of Cherish and you can share with our audience, please, what Cherish exactly is and what it’s doing and what inspired you? What was your vision to bring this to the market?
Sumit Nagpal
Awesome. I’ll introduce Cherish in very simple terms. We build advanced sensors in AI and our job, our goal is to help people like our parents, our grandparents, our kids, many of us who may be living either with health challenges or who may be aging, live more safely and with more joy, wherever they happen to be. So we’re using advanced AI and advanced sensors to go help solve some of the challenges that our populations face.
It’s very clear that healthcare’s coming home. The pandemic has shown us many of the types of health and care services that we can now deliver to people at home that we simply wouldn’t have had the incentive to put in place before the pandemic.
What’s been super obvious to me, however, is that if we can reliably predict rising risk for people before that becomes an ambulance ride, an emergency room admission, a hospitalization, we can take out a whole lot of cost to our economies and also take out a whole lot hardship for our loved ones that they go through when they wind up in these situations.
Being able to do this at scale, at a scale that matters, is the motivation behind Cherish.
Maria Palombini
Absolutely. I think that is big area of interest for us here at the Healthcare Life Science practice is figuring out how these technologies can support this growing aging population, as well as the ability to reach the unreachable. You’ve done quite a bit of work in the digital health sector, talking about advanced AI, advanced sensors. Maybe you could share a little bit with our audience, some of your experience, your research, working with different groups and tech gurus, just to give a little bit of firsthand, what you see in this space and where you see the difference and where maybe potentially see it going.
Sumit Nagpal
AI and ML are really helping create a bit of a revolution in what we can do with technology. Our teams just tying this back to the reason we exist, our teams are working to blend all sorts of signal about everyday life. As people go about their day, make sense of that signal to predict the kinds of rising risk that can help keep people out of ambulances and emergency rooms.
Today, there are rules and algorithms that let us deal effectively with some of the signal, some of the time. An elevated heart rate or a depressed respiration rate, being able to use evidence-based rules, being able to raise an alarm someplace. That’s very well understood. There are evidence-based pathways to work with such data, but when you start deploying the kinds of sensors you find on autonomous vehicles to understand how people are doing.
On the one hand, the scale and the kinds of things you can address just mushroom in scope in size. On the other hand, the complexity as well, mushrooms in scope in size. It’s a whole new ballgame with so much more signal to make sense of that these traditional techniques no longer suffice. So we’re doing things with machine learning in weeks and months, that would take literally years to decipher out of the raw data that these sensors produce.
And these capabilities are auto catalytic. They build upon themselves. It all ultimately translates into time to market and scale for health applications that help real people stay healthy or get care before they become more sick. It all translates into lower cost through preventive maintenance rather than expensive repair jobs.
It all translates into reduced hardship. AI and ML are absolutely indispensable to enable these changes at a meaningful scale.
Maria Palombini
Very pertinent point. I think we hear this quite a bit. We’re seeing all this AI at the edge and all these different devices and there’s just real growth and trends towards there. So I’m very excited to hear that you have seen that and continue to experience it as well.
There’s this aging population that potentially might not have access to caregivers that wanna still be independent, or they might actually be in a living facility, but still need care and there might not be enough human resource to support them. Can you give some examples or case studies in the work you’re doing with Cherish to say this is potentially an accepted way or a very pragmatic way to start supporting that aging population.
Sumit Nagpal
Great question. It’s the essence of what we’re focused on. While I’m not ready to share all the details of what we’re doing, what I can say is that our technology will extend the ability to monitor people’s health and safety into what we call all of life, rather than just in those episodes when you’re in a hospital or when you’re admitted, into all of life.
Just imagine if there had been something in people’s homes or in the places where they live nursing homes, care homes, senior living facilities, at the start of COVID something that detected rising heart rate with depressed respiration. Just those two things, that just happened to work all the time that people didn’t have to remember to wear that people didn’t have to remember to turn on or charge. It was just there, present in people’s lives. That would’ve been the Canary in the coal mine to raise an alarm that so many of our parents and grandparents were not okay. That they had come into contact with the disease. We could have seen this early and taken action sooner, perhaps started treatment where they live before they wound up in ICUs and ventilators or worse.
The same with emerging models of care that help bring eventually hospital level care to the home. Most of these models start with an emergency admission. Someone shows up in an ambulance in an emergency room and these models then figure out rather than admitting somebody to a medical floor upstairs, how do you send them home and then actually admit them at home. Imagine if we could detect that rising risk before that ambulance ride, this happens over and over again every day. Imagine if we could prevent those ambulance rides. Imagine the impact on cost. Imagine the impact on reducing hardship, right? So that’s what we’re working on.
Maria Palombini
That’s amazing. I imagine someone hears that message and it’ll be very welcoming for sure.
When we talk about new technologies, there’s always a multi-generational impact, right? You say, well, new technologies is conducive to this age generation. Usually the older generation, you have a, let’s call it a mixed bag. Some who are very technology savvy and some who like, I will say, my parents didn’t even know how to turn on a computer and there’s no such thing as a smartphone for them. So how are you guys preparing or ascertaining the landscape of that sort of aging population in trying to introduce these technologies and these opportunities to them?
Sumit Nagpal
We have a particularly unique perspective on adoption and engagement. We’re taking approach that recognizes that people don’t wake up every morning to use their digital health app or tool. They don’t wake up every morning to live in it, to keep their gadgets charged, or even to keep them on their bodies.
We’re taking that weakest link of the chain, that human operator and this reliance on somehow them changing their behavior completely out of the equation. People in healthcare love to talk about engagement, patient engagement, in particular. I could tell you anecdotes about people who’ve come from other, very consumer centric industries into healthcare and they’ve asked me, what is this patient engagement these people speak of? We think that’s exactly the wrong thing to try to achieve. People want to live their lives with simplicity, with ease, with joy. Let’s get out of their way. Let’s give them tools that help rather than make them feel bad because they’re not using them or don’t want to use them.
Let’s get outta their way with tools that just work behind the scenes, keeping them safe, raising alarm when needed. That’s how we’re going to actually solve this adoption challenge. And that doesn’t apply just to older people. It applies to everybody. The number of applications that people download and then stop using within days or weeks, we’ve read lots and lots of anecdotal studies about this. We think that the way to address this problem, which really works for all are one of our principles is designed for all. And one of the basic rules of that basic guidelines behind that principle is to not rely on behavior, change, not rely on people in different demographics, using different techniques to be engaged.
We think that’s a slippery slope and a recipe for failure. I hope that gives you a sense of at least our thinking around this whole issue of adoption. We think that older people not being able to adopt technology is a red herring. We think that technology has not been good enough for them to make it a part of their lives.
Maria Palombini
Very good point. We actually just did a telehealth competition on this topic. You know, the ability to say that it’s innovation, but it has to be innovation designed for all. And that was really the idea there. There’s another area that I know about Cherish, just reading some of the testimonials on your website.
One of the areas where we’re looking into is this explosive growth of mental health, digital therapeutics. A lot of it being driven from a commercial sort of, you know, go to this app site and just download it. And we’ll help you with whatever potential disorder you might have from anxiety to post traumatic stress disorder.
But I also know your tool can also help that group. How do you differentiate from what you might be seeing everywhere on TV or on the internet now, and really be able to say, we have a trusted tool that can really support, this population of patients.
Sumit Nagpal
So in this area, I’ll say that we’re still in what I would describe as early days, we see some really incredible applications coming again, without making people work for them or do unnatural things, absolutely not requiring any behavior change. And that behavior change goes all the way to remembering, to plug in something or charge something or wear something. All those things get in the way. We hope to be able to tell you more about this over the next year or two, but to give you a sense of direction, we expect to be able to pick up changes in mood, changes in people’s mental state.
Grandpa’s getting depressed. He’s taking longer and longer to get outta bed every morning. Those kinds of things. We expect to be able to do that over the next few years. And we think that can have a profound impact on them getting them the help they may need getting them the support from their families that may need before that becomes a much worse condition.
Maria Palombini
I’m fascinated by this and I really hope you keep us in the loop on it because we have definitely been looking at some of the nuances around digital mental health therapeutics for the elder population. And this is an area we’re covering in one of our industry connections program called Ethical Data Assurance for Digital Mental Healthcare.
So definitely very interested to see how that progresses for sure. So Sumit, when I say AI for Good Medicine, what comes to your mind and why?
Sumit Nagpal
First thing that pops in my mind is freedom because the pace at which healthcare is evolving to create the kinds of solutions I’m describing is just too slow.
Someone I met more than a decade ago, who’s become both a mentor and a friend to me, he was in the audience when I presented an approach to joining up medical records across a city region, this was in the UK. Then another gentleman talked about another approach. He was as sincere as I was, but he followed his thoughts with a comment that he said, you know, it’s going to take us the rest of this decade, the next decade to put this in place.
And this gentleman who’s become, my friend said, so who’s gonna take care of us and our parents in the meantime, there’s this sense of urgency. And AI is an essential part to answering his question, which is really about getting solutions to market in the here and now. It’s about our parents, our grandparents, our kids, our families.
Those are the people we’re talking about here. And that sense of urgency is what drives us. And so freedom is the ultimate impact of being able to bring these kinds of technologies into the service of their daily lives. I think that word really sums it up.
Maria Palombini
And that would be a first because I’ve heard many different answers to that question and I really appreciate the insight behind that. And I think it’ll give everybody listening, something really to think about.
We all know the healthcare inequity challenge we face globally, obviously, exacerbated during COVID, unfortunately for those who are already disadvantaged, but some have argued that artificial intelligence and machine learning can support fairness, personalization, and inclusiveness in healthcare, really starting to cut at that inequity issue. And then others find that it actually might potentially create more inequity in the healthcare system. I think the populations you’re already starting to work, with with your platform, starts to maybe cut away at that concept, but how do you see it from your point of view?
Sumit Nagpal
I think I’m gonna answer that question with one word, which is scale, but let me take a step back. I’m on the board of an organization called HIMSS, which is the world’s largest membership body that represents health and health IT users and their suppliers. This year, our global conference, which is coming up in Orlando in two weeks, we’re gonna be devoting quite a lot of time and energy to talking about health equity.
Because we are seeing the Gulf between the haves and the have nots continue to grow. And because real biases caused by perception of race, gender, socioeconomic status, they cause real harm to real people every single day. That’s fundamentally indisputable. The scale and pervasiveness at which AI and ML can be put to use to help people is staggering.
Of course, what that means is that our training data has to reflect the diversity of our populations and not include these biases into the infrastructure. This is all quite feasible in the hands of well-meaning self-aware people, right? So I am super bullish about AI and ML, actually being able to reduce health inequity, actually spread this technology into the kinds of things that people use every single day, without them being expensive gadgets that only a few have, and the kinds of things that ultimately become just parts of the furniture and fixtures of the place you live rather than special tools and special gadgets. Again, that only if you can afford.
Maria Palombini
I think that’s a great perception. It’s just fascinating to see. Hopefully that opportunity at scale can really address some of these issues.
All right. So we have a great idea. We have a great technology. We have a great opportunity and a patient population that can utilize it, but we still find that we keep running into some kind of challenge. There’s still security lapses, or we need more open data standards, or just a lack of standards, better policy. What do you think is single most challenging part currently not addressed when it comes to the use of AI applications that continues to cause concern or uncertainty in the trust in those tools and using them, what is it? And what might be the best approach in trying to start addressing it.
Sumit Nagpal
So I saw a video recently that talked about health data interoperability standards, and it was really interesting. The stuff that they were talking about was virtually identical, the same words that were being used 25 years ago. We’re still talking about the same topics when we’re close to sending people to Mars and we’re flying rocketships into the sky, like riding a tricycle, and we’re still stuck in the old ages when it comes to these topics. I don’t think that any of this has to do with not enough standards, not the right standards or not the right public policy, et cetera.
I think those issues are actually utterly business issues and those issues will get addressed when this type of technology becomes more democratized when it becomes more ubiquitous when it becomes more available to people for themselves, rather than somebody having to supply it to them. So there are two ways I can answer your question at least.
One, this notion of engagement and adoption. A lot of people who are building solutions think that their human operators are bad users rather than designing systems that eliminate the need for requiring behavior change. Those will continue to fail. This applies to, as I’ve said, thousands of digital health apps out there today. If you design the need to support a user into an app or a tool you’re designing the app or the tool to require people to need support. That’s just how it is. These challenges transcend all such solutions. They result in AI driven tools that behave even worse. And this applies to all these other topics you mentioned as well.
The other way I can answer this question is about the special challenges that are on privacy and security created by AI and ML. There are bad things that bad actors can do with this always on data about people that are made even more creepy with AI and ML, video data in particular.
The way to address this, not requiring this data beyond the inference at the edge. Gather the data at the edge, make your inference, run your AI models there, and then void the data. Eliminate it forever is a great way to delete the entire possibility of a whole class of privacy and trust issues.
Then letting people control and only them control their own data is the other. There are some companies, very large companies in consumer space that have made that their religion, right? You control your own data. You hold the encryption key. Nobody else can get it. And then there are others who live off selling your data.
They think that there’s just the market speaks. Buyers reward companies that behave well. And I think that’s, what’s gonna drive the right solutions. It’s really that simple.
Maria Palombini
I agree with you, perceptually saying that consumer driven best practices are really important and I think they can really maybe change a market mentality for sure.
So you’ve already shared so much great insight with us. There’s so many ideas, like you said, healthcare is coming home. These are really important insights and topics for people to digest. So I’m gonna ask you, is there any final thoughts that you would like to share with our audience?
Advice for a tech entrepreneur, a young engineer on the verge of the next breakthrough, or a call to action from all the different multidisciplinary professionals listening to this podcast today.
Sumit Nagpal
So I’ll go back to something my dad said when I was probably seven years old and I didn’t quite understand what the heck he was talking about.
In a moment where I clearly frustrated him. He said, think big man. I was probably seven. I’ve tried to do that ever since. Um, so dream big. Don’t let challenges stop you, embrace them instead. Think of your journey as an ever evolving puzzle that you wake up every morning to solve rather than a burden to overcome.
It just changes how you deal with it. Use that to stay fresh inspired. And this has been so important to me find a mentor or two, I’m lucky to have many to stay grounded and inspired.
Maria Palombini
Absolutely. I think that is a very positive note, mind over matter. Well, everyone, if you wanna learn more about Cherish visit cherishhealth.com.
Many of the concepts we talked today with Sumit are addressed in various activities here at the IEEE SA Healthcare Life Science Practice. We have so many global experts, even Sumit, is part of our Transforming the Telehealth Paradigm incubator program. Working together, trying to explore, collaborate, look for all these different types of solutions that are needed to continue to open the doors for innovation. You can find out about all of our practice opportunities programs at ieeesa.org/hls.
If you like this podcast, please share it with your colleagues on social media. You can use the hashtag #IEEEHLS or tag us on Twitter @ieeesa or on LinkedIn @IEEE Standards Association. This is the way we get our word out about our podcast interviews to share the insights of our volunteers, our guests, with the rest of the world.
I wanna thank you Samit for joining us today. You have been very inspirational and insightful.
Sumit Nagpal
Thank you so much for having me again.
Maria Palombini
And I wanna thank you the audience for being with us, and I wanna wish you all to continue to stay safe and well until next time.
Listen On The Go
About the Host
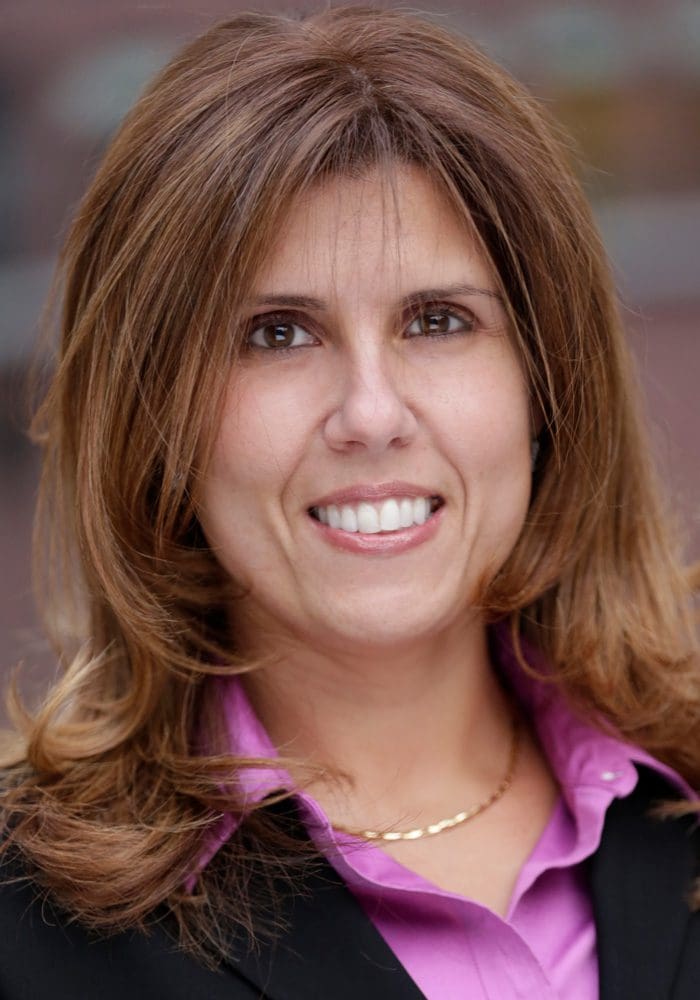
Maria Palombini
Director, IEEE SA Healthcare & Life Sciences
As the leader of IEEE SA Healthcare & Life Sciences, Maria works with a global community of multi-disciplinary stakeholder volunteers who are committed to establishing trust and validation in tools and technologies that will change the approach from supply-driven to patient-driven quality of care for all. Her work advocates for a patient-centered healthcare system focused on targeted research, accurate diagnosis, and efficacious delivery of care to realize the promise of precision medicine.
Get Involved
If you would like to participate as a guest, underwrite the series, or share topic ideas, please email Maria Palombini.
Receive Updates
Stay up-to-date on new releases and related activities by subscribing to IEEE SA Healthcare & Life Sciences.
IEEE does not endorse or financially support any of the products or services mentioned by or affiliated with our guest experts in this podcast.